Three approaches to deploying generative AI for brands and corporations.
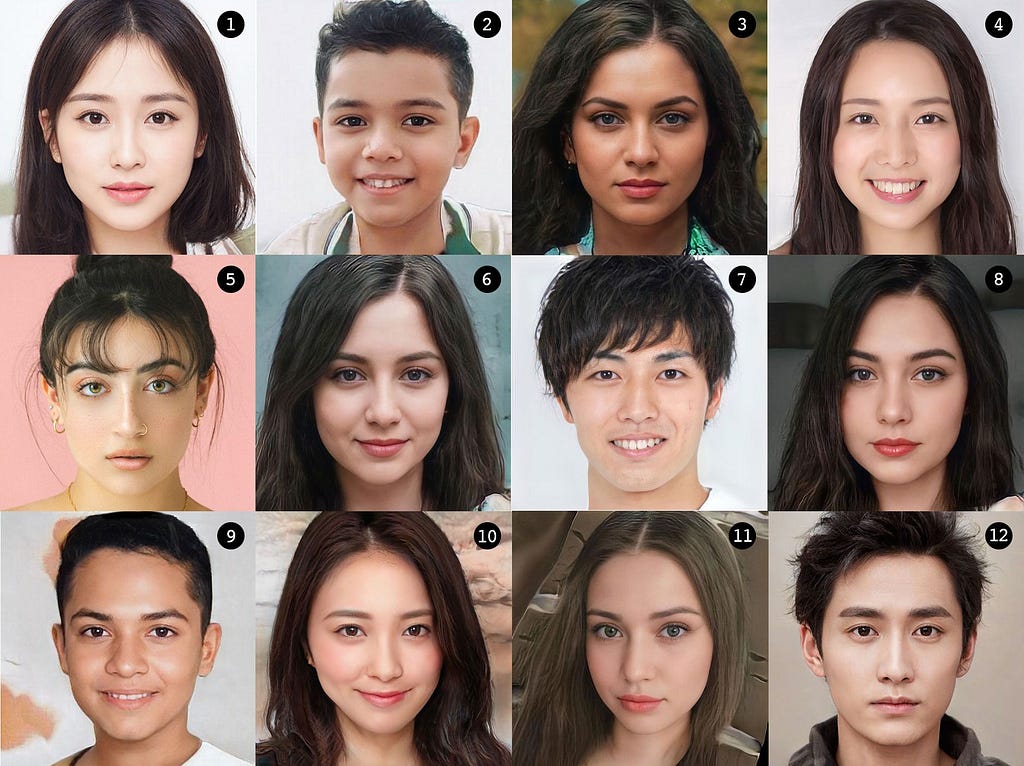
History rhymes
This isn’t a trick question: 11 out of 12 in the image above are generated by AI. Which one is the real person?
I posed this quiz as an icebreaker for an executive meeting at a major, international brand. The CEO of this company had asked me to give a talk to their 20+ top executives about where AI is today and where it could go.
No one got it right.
After the talk at this Fortune 500 company, one executive commented, showing skepticism toward what AI could do for the company, its employees, and its business.
“Are we so busy that we need to rely on AI?”
Oh boy. My icebreaker didn’t land, did it? I was startled by this comment a little. That quickly subsided.
I’ve seen this script. History doesn’t necessarily repeat itself, but it rhymes.
Twenty-odd years ago, when I started my career in digital design, corporate executives, particularly in marketing, had similar skeptical stances towards digital. Even in the mid to late 2000s when there was a growing and healthy-size audience online, some marketers were still dismissive.
I was a “digital” creative director back then at a creative agency. Anyone above 40 in the industry would remember that our “digital stuff” used to be given a graveyard shift in a presentation deck. In one client meeting with a CMO, after the “traditional work” was presented over 45 minutes, it was the turn of the digital team.
“OK, digital. Cheap and quick stuff,” said the CMO chuckling.
Adopting early
Among the non-technology brands that I work with and what I hear from others, it’s a mix of elated embrace of and uneven interpretation of AI. Many of the brands that have embraced AI, particularly generative AI, have done so more for the sake of PR (like this, this, and this). To be clear and fair, this is not my criticism or cynicism. At least they are experimenting and experimentation can lead to unexpectedly good outcomes.
The others that haven’t are cautious because of data security, plagiarism, fake work, and unpredictable hallucinations. This is also understandable as these issues can have serious liability consequences.
There is general confusion around how to start and where to deploy AI, even though they know they should.
Embracing new technologies early has its upside as we’ve seen in the case of NFTs and the metaverse in the recent few years. At the same time, their success can descend as quickly as they ascend. Andreessen Horowitz, aka a16z, handsomely made billions of dollars from the early investment they made in cryptocurrency, NFT, and Web 3.0. At the same time, we all know that the market value of the same sector plunged by 90% in the last 18 months.
Making early bets and winning big, or else, is the business a16z is in. Most brands aren’t in that business.
The evolution of AI
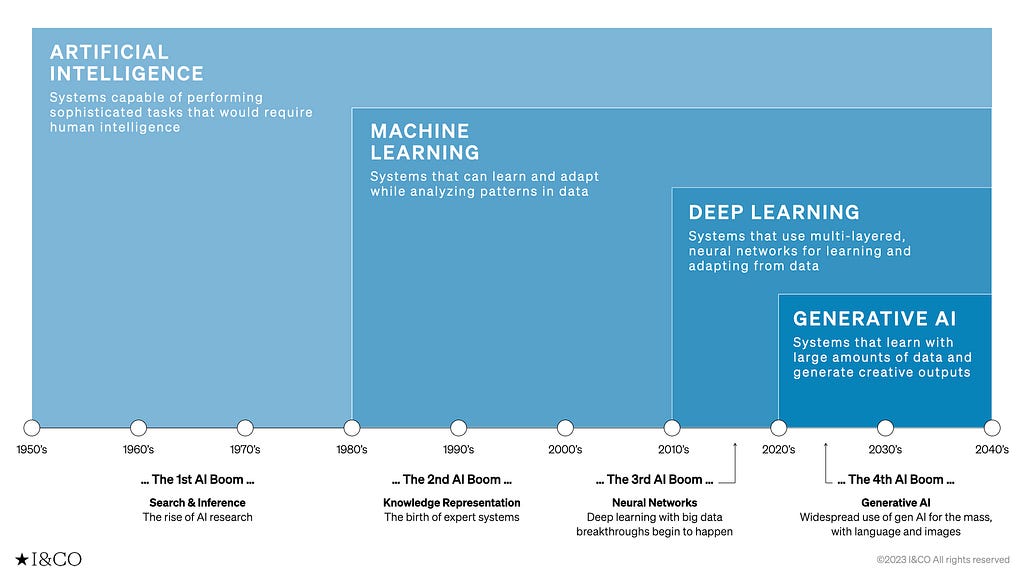
In 1955, then-29-year-old engineer Jonathan McCarthy used the term “artificial intelligence” in his writing. AI has been around the block for quite a while in various forms.
Up until several years ago, there was a varying degree of predictions around artificial general intelligence, or AGI-AI that is general enough that perform a wide range of tasks like a human instead of a specific one-and the possible timing of it becoming a reality. Some AI experts predicted it would be around 2030, others said 2050, and few predicted never.
ChatGPT, other LLM systems, and other generative AI tools may change that timeline more drastically than the experts thought.
Here’s a quick look at the several waves of AI evolution:
1st boom: 1950s — 1980s
- Artificial Intelligence: Systems capable of performing sophisticated tasks that would require human intelligence
2nd boom: 1980s — 2010s
- Machine Learning: Systems that can learn and adapt while analyzing patterns in data
3rd boom: 2010s — 2020s
- Deep Learning: Systems that use multi-layered, neural networks for learning and adapting from data
4th boom: 2020s — beyond
- Generative AI: Systems that learn with large amounts of data and generate creative outputs
The current AI situation is chaotic. However, as was the case with personal computers, the Internet, and smartphones, it is gradually converging.
It is an exciting time. There are numerous tutorials, classes, and self-proclaimed experts and gurus as well as optimists, pessimists, and skeptics. At an individual level, it is useful to start playing with the tools to see where it leads us. However, at a corporate level, it is necessary to frame the challenge we face in a strategic way to make some sense of the way forward.
I, with the help of my team, organize the current situation in the following framework: “Operation AI,” “Creation AI,” and “Transformation AI,” each of which will afford a benefit
Operation AI: A tool for improving efficiency to achieve “New Speed”
Creation AI: A tool that raises the average and elevates us to a “New Standard”
Transformation AI: A tool that transforms the status quo and allows us to reach “New Scale”
1. Operation AI
When talking about AI today, the most obvious and immediate area of deployment for corporations is operational. It gives us ways to gain efficiency in our daily tasks.
Such tasks include:
- Transcription
- Interpretation and translation
- Preparation of meeting minutes
- Text summarization
- Creation of slides, materials, manuals, etc.
- Sending emails
- Data analysis
Here’s one example of an operational use of generative AI to supposedly improve productivity:
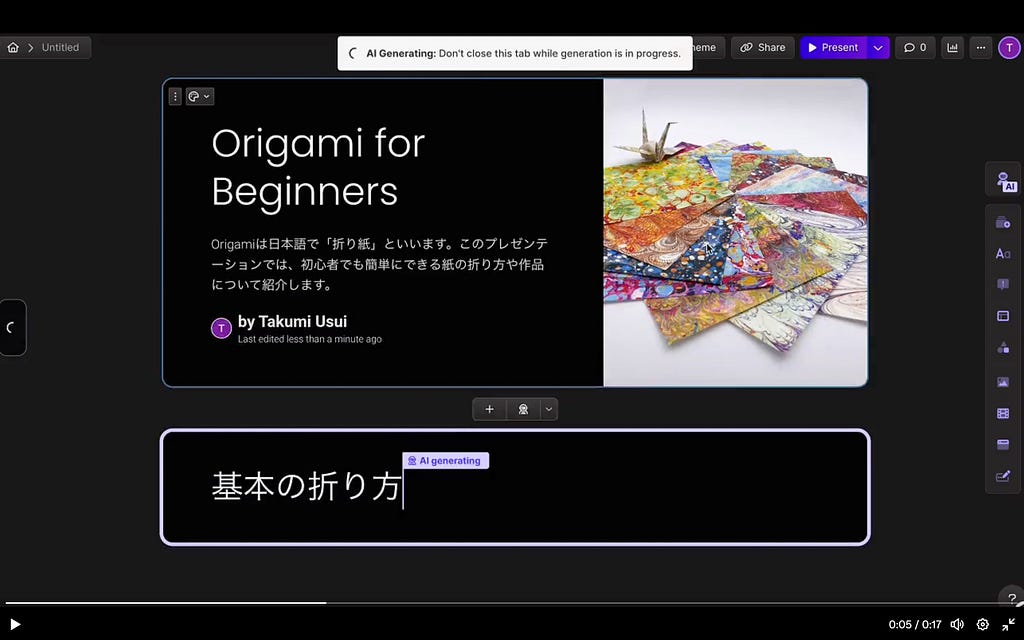
Gamma: AI for presenting ideas, beautifully
One of the Achilles heel challenges of generative AI is that it requires a massive amount of data. Big Tech companies like Google and Microsoft are at a major advantage as they have access to data and millions, if not billions, of people already using their productivity tools such as Word/Docs PowerPoint/Slide, and Excel/Sheet. Generative AI can be incorporated into this seamlessly.
https://medium.com/media/3c2dbd59ddb8765c5a3efb2aa18ff32f/href
If there are tasks that we are repeating, they probably can be replaced by AI.
Speaking of repeating tasks, image generation for e-commerce is one such area. Retail companies outsource that work to other more cost-effective vendors but that will be changing, if not already.
TryOneDiffusion, an initiative from the University of Washington/Google Research team, shows great and convincing promise for the very near future of an aspect of shopping.
What this tool does is to take two images-one, a person and the other, a garment worn by another person-and “diffuse” those two to “generate a visualization of how the garment might look on the input person.”
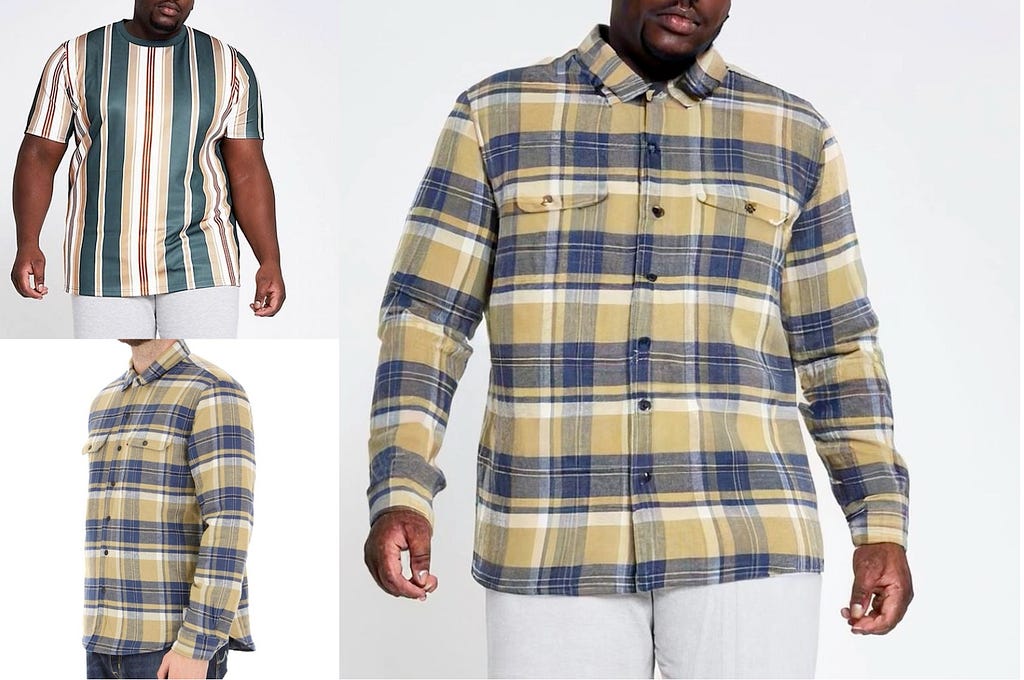
What this would allow retail brands to do is not just generate images of the products on the fly on various models but also what the product might look like on the customer.
Operation AI = New Speed
Operation AI brings “New Speed,” the kind of efficiency and productivity to us. The strength of AI is not that it gives correct answers, but that it gives us speed. Everything can be executed at high speed. However, that does not mean that it’s correct and humans do need to intervene to confirm the output.
2. Creation AI
Writing and visualizing used to be a skill reserved for humans. Not only did that change drastically with generative AI, but it’s also improving exponentially, as we’ve all seen in a comparison visual like this:
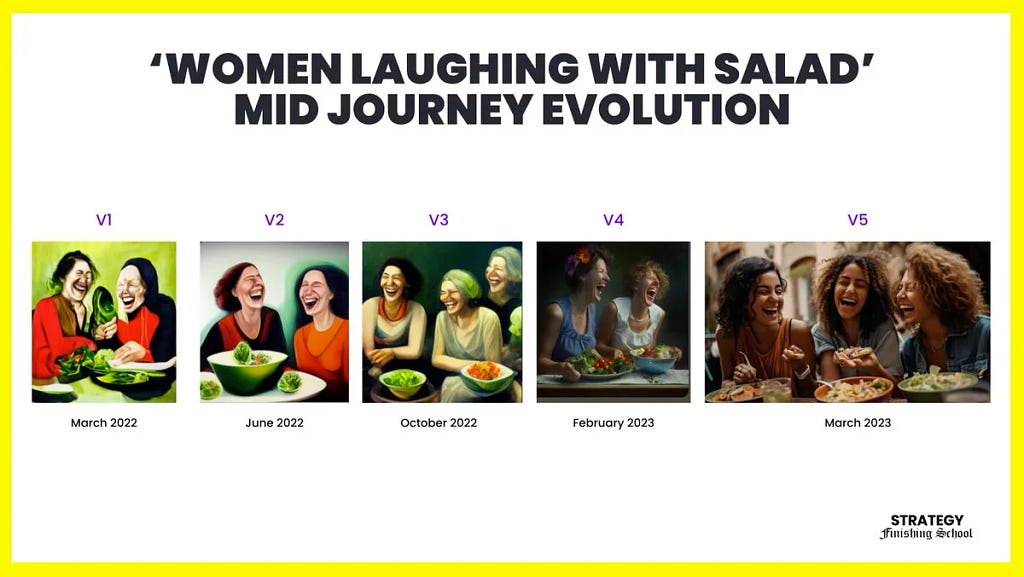
In addition, in understanding the current state of generative AI as it relates to creating various forms of content, this table from Sequoia Capital is useful in quickly getting a sense of where we are and where we are headed. This is, however, from September 2022, and given the speed at which AI is progressing, some of these predictions may be outdated.
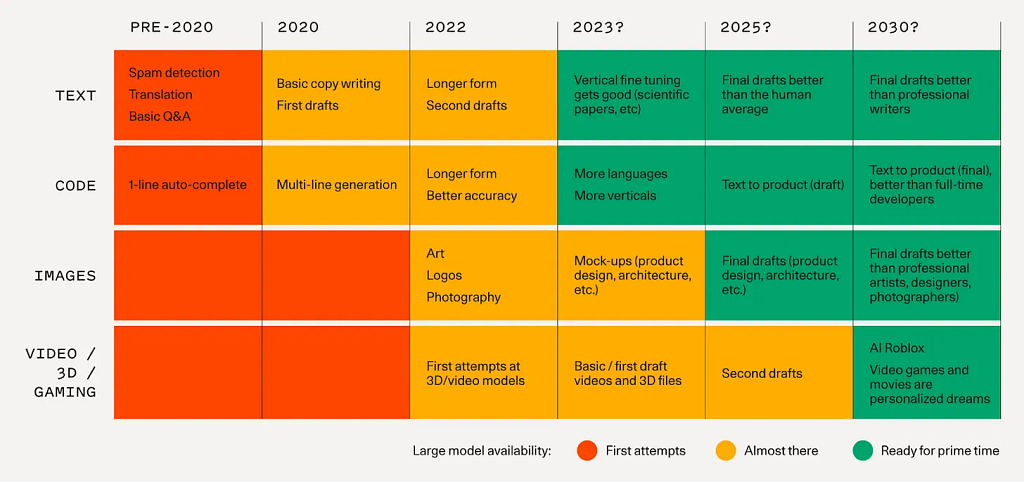
Some predict that the majority of content online will be synthetic shortly. That might also be true in the physical world sooner rather than later.
The *airegan line of sneakers is an experiment by independent designers and artists for now using generative AI to create endless options. We can easily imagine that there is already a new crop of brands we haven’t heard of that leverage AI to generate numerous designs based on what’s trending online.
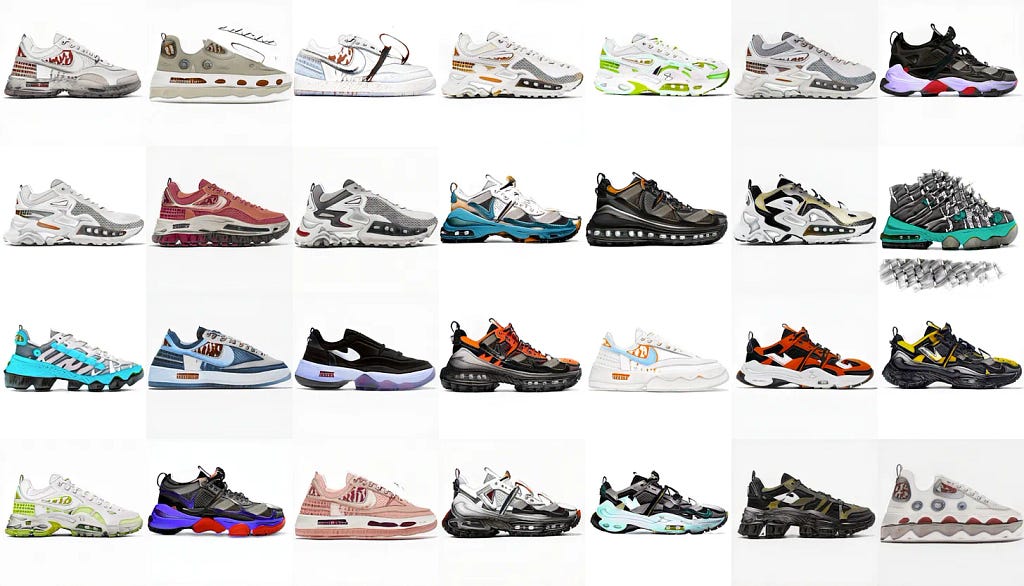
If quantity and variety are the selling point, like in fast fashion, Creation AI could prove helpful.
The next Shein is probably on its way.
The majority of generative AI tools tend to skew towards Operation AI rather than Creation AI. That is, they promise to increase efficiency rather than effectiveness. Taplio, a social media content creation and planning tool focused on LinkedIn is one such case I’ve seen. Its authoring tool, based on your original writing, suggests how to rewrite for better hooks and better engagements, and gives specific reasons why the rewrites it gives would be more effective, allowing the author to improve as they use the tool.
A recent collaboration between Claire Silver, an AI collaborative artist, and another fellow digital artist Emi Kusano is an attempt at imagining the new possibilities. One no longer needs to have design skills to be a designer. Silver says, “The barrier of skill is swept away. In this evolving era, taste is the new skill.” Silver and Kusano on their own may not have been able to create these images. That barrier is now indeed gone.
Jonas Peterson is another photographer who turned to AI recently for his work. Peterson’s strength as an image maker is the narrative he creates behind his work. His previous line of work was wedding photography, but if you look at his earlier, wedding photography work, it has a distinct feel that sets it apart from other run-of-the-mill photography out there. His photographs look like scenes from movies-which tools like Midjourney are decently skilled at producing based on prompts. He’s now using his taste to apply to AI art.
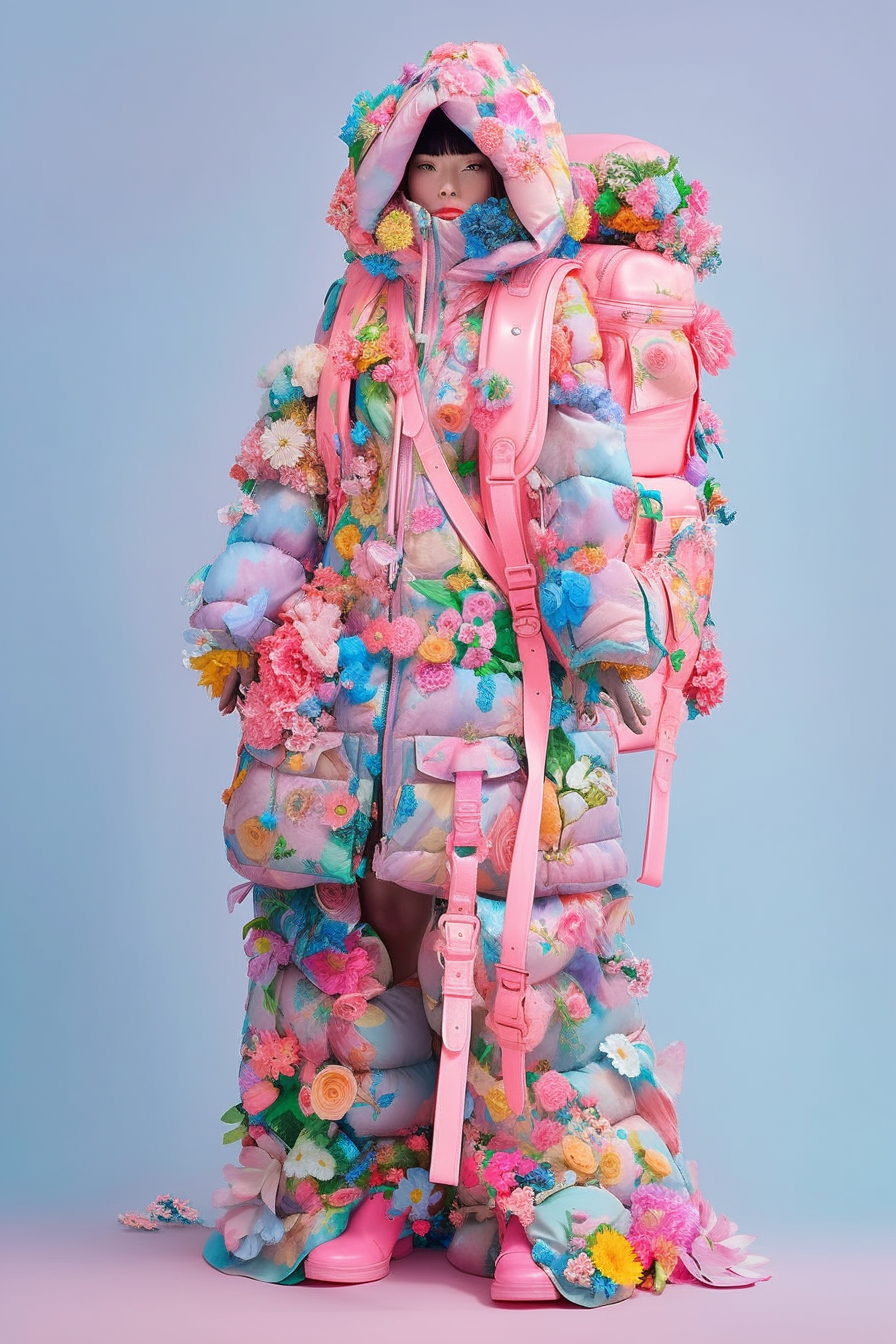
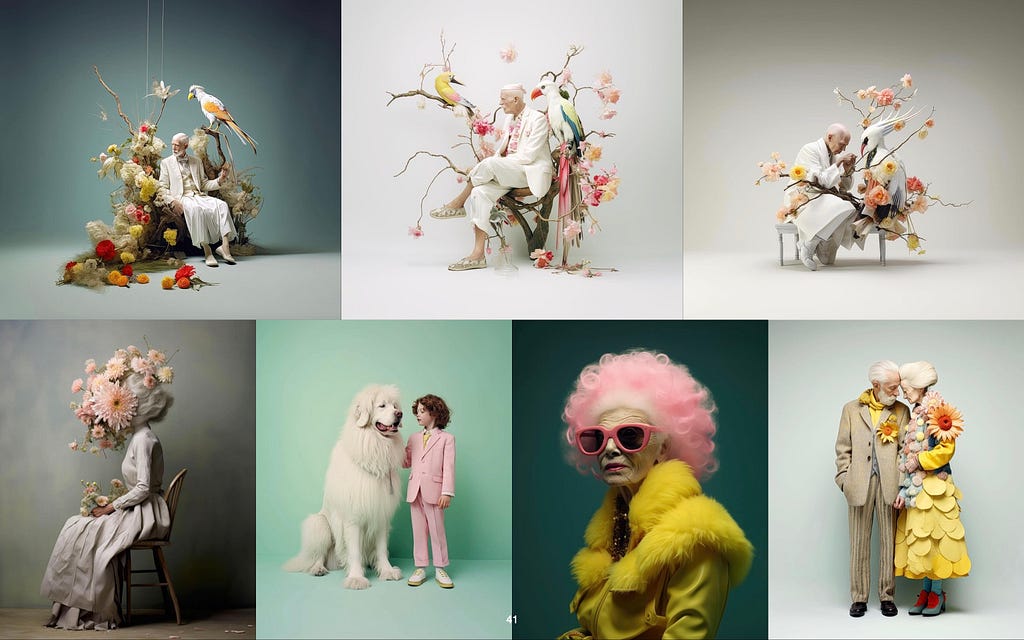
Creation AI = New Standard
Creation AI raises the bar, creating a “New Standard.” Creation AI’s strength is not in generating ideas, but in raising the average that humans can create. It democratizes and de-industrializes creativity.
3. Transformation AI
The opportunity that generative AI affords us is to rethink how we can operate our businesses and transform them.
Throughout the centuries, we have seen transformations in media and how we relate to the world around us.
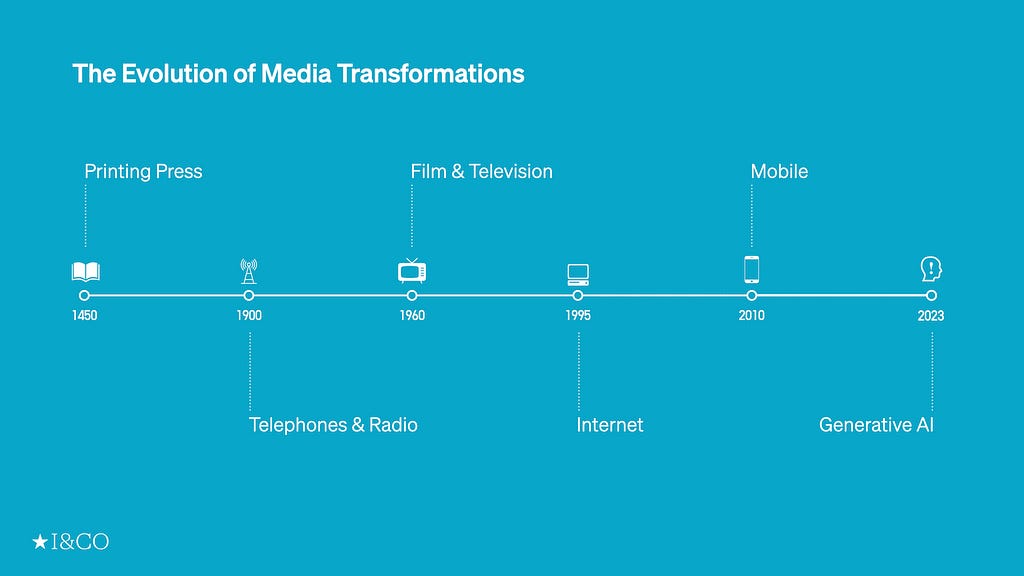
What this evolution brings to brands and corporations is that, in addition to the New Speed and the New Standard, it also allows us to reach the kind of reach and scale never possible before.
In the Age of the Internet and Mobile, technology allowed not only corporations to connect with individuals, we were able to connect with brands on our terms. What each of us saw from a brand, however, was mostly the same. That’s because the communication was either mass-produced, templated, or pattern-matched.
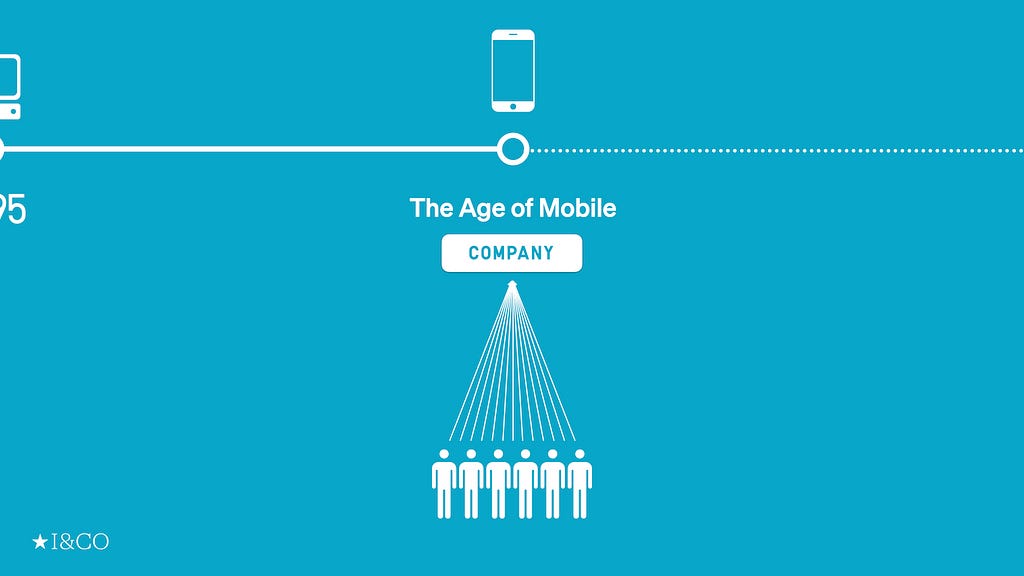
This can change radically in the age of AI.
The representation of a brand no longer needs to be singular. A brand can-and should- have one, consistent voice, but with it, it can also cater to the individuals separately and specifically.
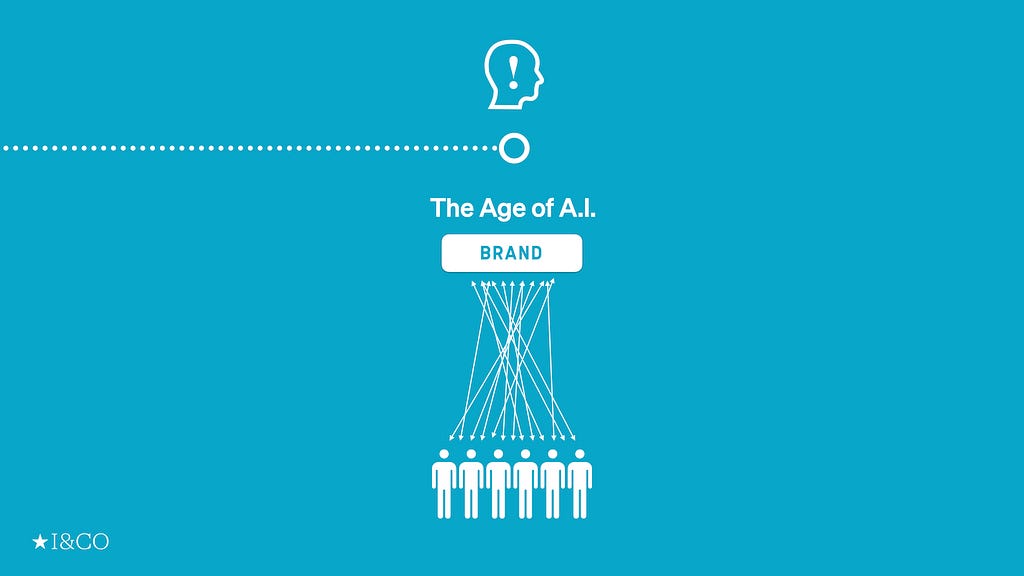
Take Hybe, the Korean entertainment juggernaut behind enormously successful BTS and other K-pop groups, as an example. They announced the first single “Masquerade” from MIDNATT, a new artist that the label is backing. What was new and unique about this debut song is that it was produced in six different languages: Korean, English, Japanese, Chinese, Vietnamese, and Spanish.
https://medium.com/media/e8401e91c1fa786df68f8788475f8469/href
It’s a good example of a partnership between humans and machines to achieve reach. By releasing the song in multiple languages, Hybe is enabling the kind of scale for its artists that wasn’t possible a few years ago.
This isn’t specific to the individuals, and it probably doesn’t and shouldn’t be. A song from an artist is something we share and we aren’t looking for some hyper-personalization.
Let’s take this another notch by the thousands.
During the UEFA Champions League Final, Adidas created a fan experience by transforming football legend Alessandro Del Piero into their personal newscaster and commentator. Using WhatsApp AI, fans could chat directly with Del Piero and they would receive LIVE updates and replies instantly from the star himself, or the AI version of Del Piero. It was at a scale that was never possible before: over 44,000 messages in 10 seconds.
https://medium.com/media/06e35c5b2ac79bbe1e013aff87e4b46c/href
The idea of personalization has been around for years now. However, personalization executions have mostly been about serving pre-generated content to the individual user based on the tracking of their previous patterns.
Similarly, Chris Do, a design entrepreneur with more than a million followers on social media who helps designers become better business people, is creating an AI version of himself to allow him and his team to serve a much bigger audience with a credible replica of Chris himself.
This type of deployment of generative AI makes true personalization possible in that it’s not based on the user’s prior behavior or engagements. In addition, AI makes it possible to tailor that unique interaction to mass and scale instantly like never before.
Transformation AI = New Scale
Transformation AI can give us the kind of reach, “New Scale” by allowing us to do more with less. Transformation AI’s potential is to help us grow our business without growing our organization significantly. It scales our reach way beyond our individual as well as organizational capability. It could transform the way we manage our business.
The answer is…
Back to the icebreaker quiz: Most people think #7 is the real person, followed by #4. The answer is #5. The tell is her ears and earrings. For generative AI, ears are, similarly to the fingers, apparently difficult to render. If you look at #5, her ears and earrings are accurately and realistically shown. Well, it’s a real photograph.
This image is courtesy of Imagenavi INAI Model, a Japanese imaging company that started offering a virtual modeling service using AI to replace human models with generated models. The real photo is by Jimmy Fermin.
I also asked at the aforementioned meeting, other than ChatGPT, if anyone had tried using generative AI tools such as Midjourney, Stable Diffusion, or others. Most hadn’t and I don’t blame them. The interfaces of some of these tools are still pretty atrocious.
One of the issues of newer technologies like VR and the metaverse that’s stopping mass adoption, I believe, is the interface. For them to become widely adopted, the interface needs to be so simple, like ChatGPT or the iPhone, that anyone can use it without manuals or tutorials.
Unlike the metaverse or NFTs, generative AI is here to stay. Enough applications of generative AI are becoming available literally on a daily basis. In sum, the three approaches to deploying generative AI for brands and corporations are:
Operation AI: A tool for improving efficiency to achieve “ New Speed “
Creation AI: A tool that raises the average and elevates us to a “ New Standard “
Transformation AI: A tool that transforms the status quo and allows us to reach “ New Scale “
Having said all of this, I do sympathize with the executive’s skepticism, at least partially. As much as I do rely on ChatGPT, Claude.ai, Midjourney, and other AI tools for various tasks throughout the day, I—and not AI—am still writing this newsletter entirely by myself, occasionally with some help from other humans at my company.
If you knew it was being written by ChatGPT, would you still read it?
Special credits & thanks to I&CO’s Daiki Kanayama, Takashi Mazawa, Riho Nishimoto, and Shohei Kubota for the research
Thanks for reading. If you like what you read, please subscribe to the The Intersection newsletter.
Originally published at https://reiinamoto.substack.com.
How to AI was originally published in UX Collective on Medium, where people are continuing the conversation by highlighting and responding to this story.