Summary:
Convenience sampling is quick and cost-effective for UX research, but probability sampling is better when you need representative and generalizable data.
When conducting UX research, choosing the right sampling method can ensure that your findings are both useful and reliable. Most UX practitioners use a sampling method called convenience sampling: recruiting participants based on ease of access. For many UX studies, this approach is fine — it’s fast, cost-effective, and sufficient for identifying major usability issues and patterns in user behavior. However, a more rigorous sampling method called probability sampling should be considered if you need higher confidence that your findings accurately represent a broader user population.
Convenience Sampling
Since many UX practitioners use convenience sampling, it’s important to understand what it entails.
Convenience sampling refers to the nonrandom selection of study participants who are readily available and convenient to access.
Nonrandom selection means that study participants are chosen on purpose or by convenience rather than by chance, which means not everyone in the target population has an equal chance of being selected.
Examples of convenience sampling in UX research include:
- Sending out a call for participants using the company’s email listserv or social media channels
- Using a company’s research-participant database to invite people who have opted in to participate in studies
- Using a user-research panel such as User Interviews’ or UserTesting.com’s panels
- Intercepting users on your site and inviting them to participate in a research study
In defining convenience sampling, there are several key considerations to keep in mind:
- The use of inclusion criteria does not preclude convenience sampling. A convenience sample may be restricted by the study’s inclusion criteria (such as including only participants with a certain occupation or who have not recently participated in a research study), but it still remains a convenience sample if recruitment is nonrandom and driven by ease of access to the participants.
- Certain types of convenience sampling will be more biasing than others and should be avoided as much as possible. For instance, testing with coworkers is generally not recommended, as they may be too familiar with the product or feel reluctant to provide honest feedback.
- Quotas do not equal randomness: Even if you set quotas for your convenience sample (say, recruiting 5 users per age group) the process still remains nonrandom. This means that, while your population segments might be accurately represented in your sample, hidden biases (such as emotional state, cultural influences, or interest in study participation) can still skew the results.
Probability Sampling
Probability sampling refers to a method of study-participant selection in which every user in your target population has a known, nonzero chance of being selected.
Probability sampling is based on random selection, which ensures that everyone in the population has a known probability of being included in the study. This probability can be equal for all users (e.g., every user has a 10% chance of being selected), or may vary based on predefined criteria (e.g., each mobile user has a 12% chance of being selected, while each desktop user has a 20% chance of being selected). Its power lies in balancing unmeasured differences across participants, such as emotional and psychological states, cultural influences, implicit biases, and personality traits. Because researchers can’t measure or control for every variable affecting study outcomes, random selection helps reduce these types of overlooked biases.
Probability sampling allows you to be more confident that your study findings are generalizable to the larger user population by reducing selection bias and increasing external validity.
Here’s an example of a probability sample: A UX research team is conducting quantitative usability testing to evaluate whether a redesigned electronic health records (EHR) system has improved usability compared to its previous version. To ensure a diverse and representative sample, the team sets participant quotas based on key demographic and professional factors, including age, medical specialty, and clinical setting. Additionally, within these quota groups, the team employs random selection to reduce potential biases from unmeasured factors, such as technological proficiency, workflow preferences, or prior experience with EHR systems.
Types of Probability Sampling
The two most common types of probability sampling in UX research are simple random sampling and stratified sampling.
Simple Random Sampling
Every individual in the population is given an equal chance of being selected, and participants are chosen randomly. This sampling method is similar to pulling names out of a hat. For instance, let’s say the target population is all active users who made at least one purchase in the past year. Using a database of user emails from the past year’s transactions, you assign a unique number to each user’s email. Then, you use a random-number generator to randomly select 100 users to participate in your study.
Stratified Sampling
The target population is divided into smaller, distinct groups based on shared characteristics, such as user expertise (beginner, intermediate, expert), device type (mobile, desktop), or geographic location (e.g., North America, Europe, Asia). Once you define these groups, you then determine whether the sample size of each group should be proportional to its size in the target population or if each group should have the same number of participants. Participants are then randomly selected from each group. This method ensures that each group is adequately covered in the sample.
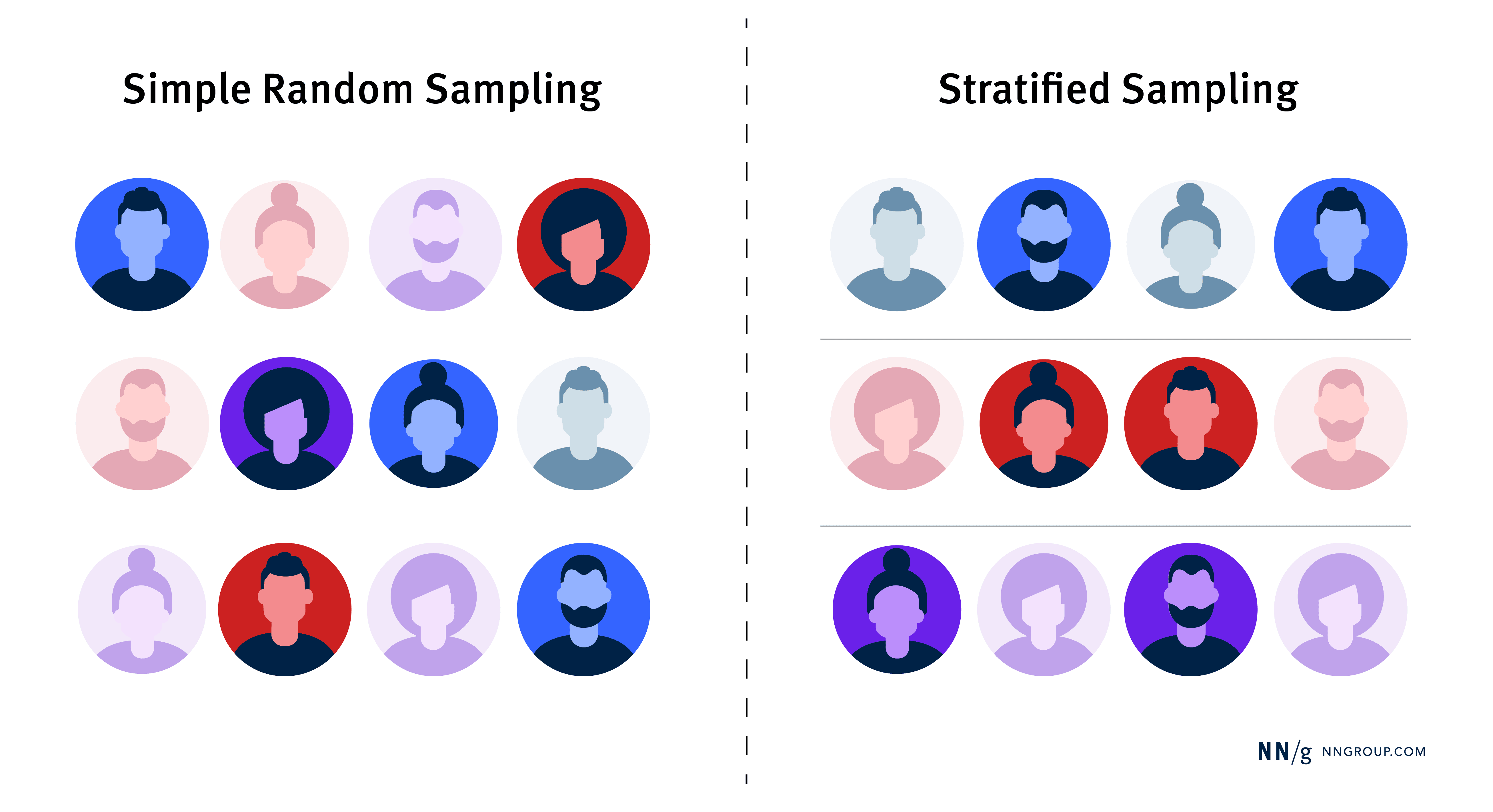
Steps to Gather a Probability Sample
Probability sampling is more complex, time-consuming, and expensive than convenience sampling. To gather a probability sample, you need to decide 4 things:
- Your target population. Before sampling, clearly define the population from which you want to draw conclusions.
- How you will obtain a complete list of your population. You’ll need a full list of everyone in your defined target population and their contact information. Without this, you can’t randomly select participants.
- The probability-sampling method. Decide whether to use simple random sampling, stratified sampling, or another type of probability-sampling method.
- The sample size: The sample size should be large enough to detect meaningful patterns and ensure that the results are reliable. We generally recommend at least 40 participants for quantitative usability testing and at least 100 participants for surveys.
When You Can Get a Complete List of Your Target Population
In some cases, you will have direct access to a well-defined group of users, which makes probability sampling feasible. Common examples include:
- Current customers with active accounts: You can sample from your customer-relationship management (CRM) database, or user-management system, assuming it includes contact information and basic usage data.
- Employees within a company: In enterprise UX research, you may have a full roster of users, making random sampling possible.
- Program participants: For example, your population of interest may be students enrolled at a university or people enrolled in a state-funded food-stamp program. In these cases, your target population is limited to existing users, and you likely have mechanisms in place to reach them (such as email or internal messaging).
When You Can’t Get a Complete List of Your Target Population
In many UX scenarios, getting a complete list of your target population may be technically impossible. For example:
- Visitors to an ecommerce website: Even if you narrow your target population to “people who visited in the past week”, you probably don’t have identifiable information for all of them, especially if they didn’t log in to a personal registered account while visiting.
- Prospective users of a new feature or product: By definition, these users don’t exist yet in your system, so you can’t obtain contact information for them. These users are much harder to identify and reach systematically.
- App users who churned or never signed up: Both groups are typically unreachable for probability sampling because you either no longer have a valid contact method (in the case of churned users) or never had it to begin with (in the case of people who never signed up).
In such cases, researchers often turn to other sampling methods that are not probability-based.
Choosing the Right Sampling Method
Here are some differences between convenience and probability samples.
Convenience Sampling | Probability Sampling | |
Sampling Method | Nonrandom: based on availability or willingness of participants | Random: every member of the population has a known chance of selection |
Bias Level | High: sample may not be representative | Low: aims to be representative of the population |
Generalizability | Limited: findings cannot be confidently applied to the broader population | High: results can be generalized to the population with high statistical confidence |
Use Cases in UX Research | Quick, iterative research, usability testing, and exploratory studies | Studying user populations at scale, making high-stakes decisions, or ensuring statistical validity in comparisons |
Cost and Time | Low cost and quicker to implement | Costly and time-consuming due to the random selection process |
Potential Issues | Selection bias, lack of representativeness | Logistical complexity |
When Convenience Sampling Is Sufficient
Convenience sampling is the default in UX research for good reason: most studies aim to find usability problems or explore user behaviors, not to make statistically rigorous generalizations about a broad population.
Use convenience sampling when:
- Your goal is to uncover usability issues. Testing with a small convenience sample in a qualitative usability test is often enough to identify major usability pain points.
- You need quick, iterative feedback. Early-stage research (such as concept testing or prototype evaluations) benefits from rapid feedback cycles with easy-to-recruit participants.
- Your research isn’t meant to predict or measure prevalence. If you’re exploring user needs, conducting qualitative interviews, or running a qualitative usability test, precise population representativeness is often unnecessary.
When to Use a Probability Sample
There are cases when relying solely on convenience sampling can lead to misleading conclusions. If you need results that reflect a broad user base with great confidence, probability sampling is a better approach.
Consider probability sampling when:
- You’re making population-level inferences. If you want to estimate how common a behavior is in the broader user population, how users in different groups compare, or what proportion of users experience a particular problem, you will need a sample that accurately represents the full population.
- Your user base is diverse, and recruitment biases could skew results. Convenience sampling often overrepresents certain groups (e.g., tech-savvy individuals, participants from certain geographic regions). If your research depends on comprehensively capturing differences across users, probability sampling ensures a fairer representation.
- Decisions carry high stakes. If findings will influence major product decisions or long-term investments, unreliable sampling can introduce major risks. Industries such as healthcare, automotive, and finance often grapple with decisions where user experience can directly impact safety, trust, and financial outcomes. Using a probability sample helps ensure that detected patterns aren’t just artifacts of sampling bias but, instead, accurately reflect the broad user population. This gives UX teams confidence that their findings can hold up in the real world.
- Your research involves statistical comparisons. Probability sampling can provide a more reliable basis for accurate comparison if you’re testing hypotheses about differences between groups.
Challenges of Probability Sampling
It’s important to note that probability sampling is rare in UX research because it can be expensive, time-consuming, and logistically complex. The challenges of probability sampling arise from the fact that it requires:
- A complete list of your population. Unlike convenience sampling, where you pull from readily available participants, probability sampling requires a well-defined list of all users in your target population and their contact information. In many UX projects, obtaining this kind of detailed user list can be unrealistic due to cost, fragmented data sources, privacy restrictions, or constantly changing user bases.
- Random selection methods. Ensuring each user has an equal chance of being chosen may require stratified or cluster-sampling techniques. While these methods can sound straightforward in theory, they can be complex to implement in practice. For instance, if you’re conducting UX research on an enterprise software tool, you may need to include users from various departments, job roles, and levels of software usage. Ensuring that all these groups are proportionally represented — and that selection within those groups is random — can be logistically complex. It may require working with client organizations or data teams to access user lists, account for varying group sizes, and ensure that no group is unintentionally excluded or overrepresented.
These challenges make probability sampling impractical for most usability studies, but potentially worthwhile for large-scale UX surveys, benchmarking studies, or A/B testing with real-world users.
Conclusion
Most UX research studies involve convenience sampling: recruiting users that are easy to access. Convenience sampling is quick, efficient, and sufficient for most small-scale qualitative research studies. While probability sampling is rare, complex, and costly, it is best suited for research that impacts critical business decisions. Consider the sampling method carefully to ensure your insights are not just informative, but also reliable and actionable.