Networked technology has networked effects. These effects are often intangibly and recursively amplified through a system when machine learning is in use. Does human-centered design still make sense when we’re designing for AI?
This post considers some of the limitations of Human Centered Design for AI tech, and considers what happens if we think about relational design for data systems instead.
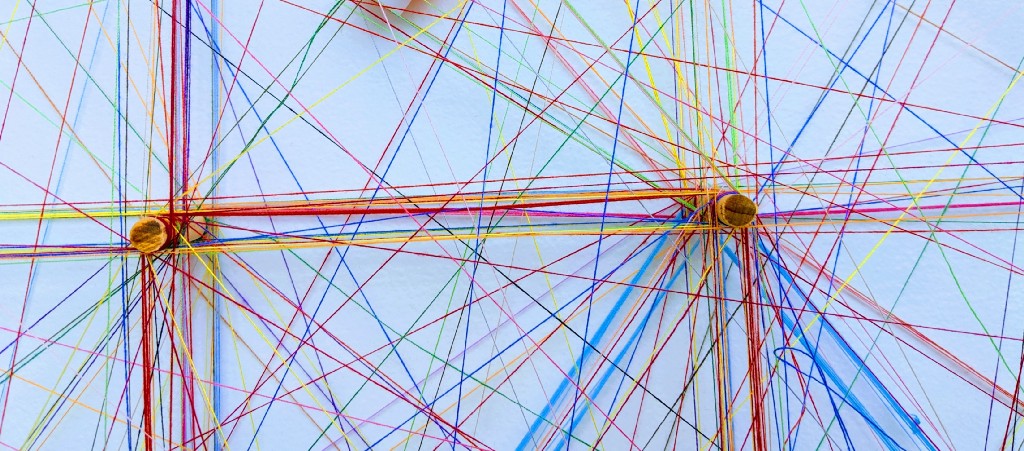
Whenever a new bit of technology comes along, new forms of design arise accordingly. The printing press brought us graphic design and typography, the production line brought us product design, and the internet brought interaction design. More recently service design has established itself to enable interactions that take place over time. Every form of design brings a new approach and way of building interesting, useful, beautiful things. Beyond that, the way we design things fundamentally informs what we build and how.
Many of the more modern forms of design share a common basis in user or human-centered design and design thinking. These methodologies aim to understand and empathise with those using the product or service and put their needs at the centre of the design. But whilst there is much to be admired in these approaches, I have been wondering if we need something new when we’re thinking about designing products that use AI.
Increasingly, HCD is part of the engineering process for building consumer-facing digital products that are underpinned by machine learning. These AI models collect and analyse user data at scale, and are increasingly sophisocated. Whilst they have affordances that require interaction and service design, the way they work different consequences to more static forms of technology. HCD has two main limitations in this context.
https://medium.com/media/83898b2255ca0269d9068e64feb2f77a/href
The first limitation is that HCD is focused on understanding and solving the problems of an individual person with elegant, scalable solutions that can be used by other individuals with similar needs. These methods conceive of humans and their needs as discrete and pretty static. But a machine learning algorithm isn’t calibrated around people as individuals. Generally speaking, ML is about the abstract analysis of atomised data and the automated discovery of rules and patterns in it.
To the type of ML model that underpins a social network for example, a ‘human’ is just a cluster of data points that has relationships with other clusters of data points. Analysing the way that relationships between the data points change, depending on different outputs they are shown, enables the algorithm can optimise itself. What this means is that our ‘user needs’ become relational to those around us in an unprecedented way.
A simple way of thinking about this is through the sociology social groupings for individuals. We all tend to have a primary group — such as our closest friends and family, a secondary group — such as wider friends and colleagues, and multiple reference groups an individual compares themself to in order to evaluate themselves and their own behaviour. Reference groups are used to determine a person’s identity, attitudes, and needs. Prior to social media, our reference groups tended to be pretty horizontal, meaning the people around us were the most influential. But now our reference groups are vertical as well as horizontal — we can probably compare our wardrobes and net worth more easily to Kim Kardashian than our own neighbours. Unsupervised learning augments what reference groups we are exposed to, and therefore our subsequent perception of need. Needs become non-linear and driven by our own ever-changing behaviour in relation to each other.
So… thinking about user needs as distinct and static might not be as useful as it used to be on that score.
https://medium.com/media/ab696a14248721f34e26008058d252bc/href
The second limitation is that most of the time users aren’t actually centered, the business model is. Donald Norman the guy credited with inventing user-centered design at Apple in 1993. He has said:
“What I teach is systems thinking and systems design and that you and nothing is an island and you have to really understand the entire story. But even our field has its boundaries and it stops at the business model.”
The boundary of the system being drawn around the business model is, of course, for practical reasons. We live in a world of systems within systems and the boundaries around social and environmental systems are fundamentally porous making it impractical to constantly be thinking bigger and bigger. This commercial reality isn’t new, but as described above, the consequenses are and relatively speaking so is the business model.
‘Frictionless’ interfaces conceal bloated back ends stuffed with dubious tracking code. This code collects personal data feeding AI models that power the surveillance economy and require a whopping server load (it is estimated that data centers account for about 2 percent of total GHG emissions). Shoshana Zuboff has pretty compellingly demonstrated that the business model has become the datafication and commodification of private personal experience (aka the surveillance economy).
Of course, responsibility for the problems arising from the tight knot of politics, power and economic policy that has led to these commercial models can’t be laid at the feet of designers. But if you’re going to use HCD to build AI that’s used commercially, you have to acknowledge that the consequence of the surveillance economy model is that the people using the products are dehumanised. As such the design methods are used in a way that becomes more extractivist than empathetic.
Most of the time, designers in commercial settings lack the resources, time, and expertise needed to do the type of participatory research that would enable them to actually engage and empathise with the people using their designs. Working within these constraints, designers are often forced to reduce people- who inevitably have messy, multiple, overlapping, historically and contextually situated identities and behaviours- are reduced to being ‘users’.
Users are often characterised as personas; two-dimensional clusters of demographics that describe a behaviour or group deemed relevant to the proposition or product. Inevitably personas are more a construct of the designer’s values imposed on groups of people than reflections of actual lived experience. These values then get encoded and amplified into people’s lives in exactly the same way that biases in data do.
Another consequence of persona-driven design is that the people between the data points simply aren’t considered. Most of the time these invisible communities are marginalised in some way; see the overwhelming evidence of how technology reinforces structural racism or Caroline Criado Perez’s work on how basically everything is designed for men. But sometimes it’s Nazi’s. Facebook probably didn’t consider white supremacists or conspiracy theorists to be their users, but it turns out they want to connect as much as anyone else.
Thinking about people in such binary terms is problematic in all cases, but particularly so for products that use AI. This need for simplification blocks the consideration of alternative perspectives or intersectional user needs and the biases that are inevitably built into the construction of the personas are then amplified through the network when released.
There is of course emerging regulation and anti-trust lawsuits on the horizon which will start to tackle some of the business model issues. But AI isn’t going anywhere so how are designers going to handle it going forward?
Machine learning seems to be supercharging our awareness that our needs are relational — and always have been. Cassie Robinson has written about ‘relational design’ describing it as ‘designing for a set of needs at different scales’.
“This kind of design acknowledges we are complex beings in relationship to one another and the wider systems of which we are a part. Health care is a good example of this — the needs of a dependent, their family, a carer, a health professional, the wider demands on the NHS — are all different but closely inter-related — no one exists in a vacuum our access to vital services is not linear, and our use of such services has a cumulative affect on the wider system.”
Can we think about relational design when we’re working with AI? One practical way of doing this could be to draw on social theory approaches like actor-network-theory (ANT). ANT is an approach that considers everything in the social and natural worlds as existing in constantly shifting networks of relationships. It describes systems as relationships between ‘actors’ be they human or ‘non human’ (processes, ideas, objects, animals, etc…). Critical to ANT is that all actors are seen as equal in their relation to one another. Donna Harraway gave this rather nice example of relational thinking:
https://medium.com/media/20316dfea4642cf6678d15f383d7a148/href
ANT maps relations that are simultaneously material (between things) and semiotic (between concepts) and assumes that many relations are both material and semiotic. This mapping means that you start looking at a system from the bottom up, picking a point to start and mapping the relations holistically. Taking all the human, technical and non-human actors in a system into account is likely to reveal more nuance than persona-driven methods. More importantly, if a designer were to do this and include themselves in this mapping, it could perhaps counter the problems raised by the invisible all-powerful designer, by situating themselves in the design.
Building on Donna Harraway’s work, Jill Rettberg has recently published this method of situated data analysis. It allows researchers to analyse how data is constructed, framed, and processed for different audiences and purposes, recognising that it isn’t possible to see the whole of a system and that it’s context-dependent. Essentially it is a practical starting point to help make the implicit power relationships that exist in platforms more explicit.
As new technologies such as AR and VR become ubiquitous, companies are likely to gain access to rich streams of observational data — from cameras affixed to the user’s headgear, but sometimes also from sonar, lidar, structured light, and time-of-flight sensors. This data will enable insights into the user’s spatial environment and individuals and objects in that environment making us even more relational than we are now. So it’s only going to become more important that designers have the option to consider the implications of this in their work.
I’m not saying that this should replace design thinking. But I am wondering if there’s value in adding these kinds of techniques to design practice when we are designing for and with AI.
Do we need a new approach for designing with AI? was originally published in UX Collective on Medium, where people are continuing the conversation by highlighting and responding to this story.