How Generative AI advances are helping shape the future of feature design, from enhancing content interaction to more informed decision-making
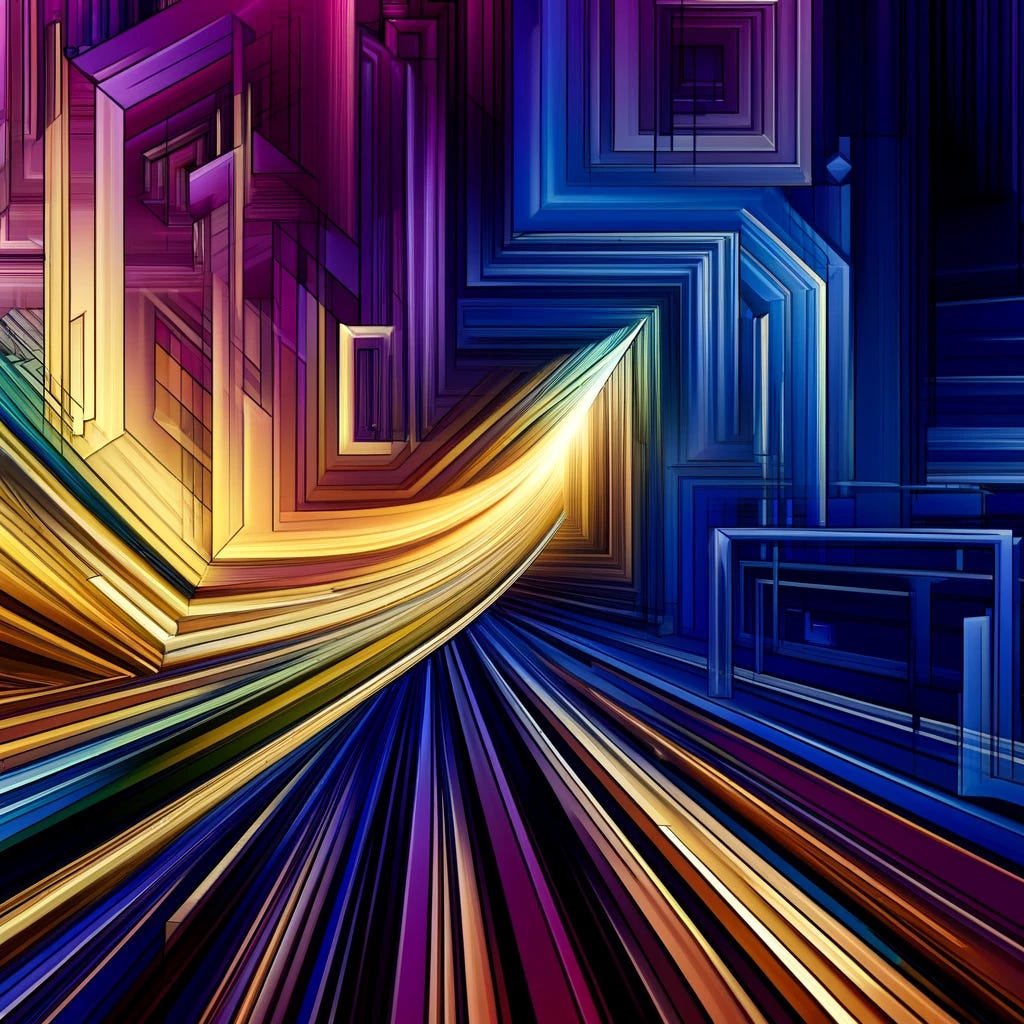
The ChatGPT revolution has been unfolding for over a year now. While the specific chatbot may not represent a seismic technical shift, the profound change in perception it has engendered within the tech community continues to send shockwaves. Initially, teams across various industries scrambled to mimic and refine the success of chatbots, leveraging the underlying models of GPT to produce solutions that, while similar to ChatGPT, were customized for specific experiences and trained on unique datasets.
As the novelty wears off and the real potential of Generative AI becomes clearer, innovative teams move beyond mere chat functions. Notion AI emerged as a beacon of how AI advancements can be integrated into traditional product features, sparking discussions and excitement about the broader applications of this technology. This was followed by companies like Grammarly and Figma, who have introduced many features that transform content creation and modification, setting a new benchmark for practical AI integration.
Fast forward to this year, and it seems every forward-thinking company has unveiled a roadmap dotted with AI-enhanced features. Product leaders are now under intense pressure to weave Generative AI into their offerings — not only to deliver genuine value but also to compete in a market where AI capabilities are fast becoming a yardstick for innovation. While chatbots and straightforward text manipulation tools remain popular for their proven value, the landscape is evolving rapidly.
Startups in every sector are pushing the boundaries of what Generative AI can achieve, from creating synthetic users for conjoint analyses to agents that can act as junior developers. This surge of creativity is expanding the Overton window, showcasing a future where traditional products are imbued with what seems like a touch of magic. All are powered by the increasingly abstracted applications of underlying LLM technologies.
Emerging AI-Enhanced Features
As I observe the ongoing evolution of Generative AI, several distinct patterns begin to crystallize. There are many ways to look at these patterns, and some have done so through more traditional design elements seen in AI Features. Others have done so by looking at patterns in the user experience. The trends I have begun to notice focus on how Generative AI is being used to make the product more effective at its intended purpose. These patterns emerge either as a cascade of mimicry — where one company’s innovation spawns a host of imitators, each tweaking the concept for their unique user bases — or as a convergence around a genuinely transformative capability of Generative AI. These standout applications are now staples on the roadmaps of many companies, representing the real value this technology brings to the table.
Recall the advent of mobile technology: initial mobile apps were often mere extensions of existing desktop services, adapted — not always gracefully — to fit the mobile format. Many were little more than clunky mobile renditions of company websites. At the time, these solutions merely extended what was already available rather than unlocking the new value potential inherent in the mobile platform.
However, as companies grew bolder in their experiments with the capabilities of handheld devices, coupled with the expansion of high-speed internet, truly innovative solutions began to emerge. A prime example is Uber, which leveraged mobile access's ubiquity to revolutionize how people summon and share rides. This breakthrough wasn’t just an adaptation but a complete reinvention, creating unprecedented value by fully harnessing the platform’s potential. Just as mobile technology found its footing by embracing its unique capabilities, the LLM revolution is now poised to transform how we interact with digital environments in equally groundbreaking ways.
Rewriting Content is a Natural Starting Point
Content Rewriting: One of the most impactful uses of LLM technology lies in content rewriting, which naturally capitalizes on these systems’ robust capabilities for generating and refining text. This application is a logical fit, helping users enhance their content while engaging with a service. Early implementations included Notion’s feature, allowing users to transform brief inputs into structured templates, and Grammarly’s expansion of its editing tools to enable comprehensive content rewrites. These integrations were a natural progression for products designed to streamline and improve written content.
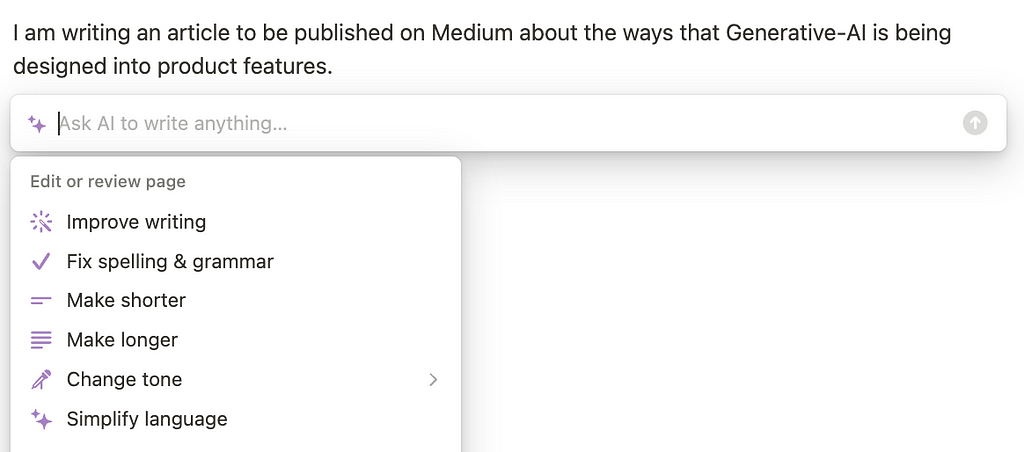
Message Personalization: As more companies have adopted this application, we see more innovative ways to assist users with rewriting content. In the sales field, both Hubspot and Salesforce have created systems to create tailored outreach to prospective customers utilizing information stored within their systems. Adding this capability creates a demand for high-quality data, further reinforcing the need to invest in building their respective CRMs. UpWork, known for matching contractors with work, has a system that enhances job postings to attract more qualified candidates. On the consumer front, Bumble recently released a tool to help create icebreaker messages.
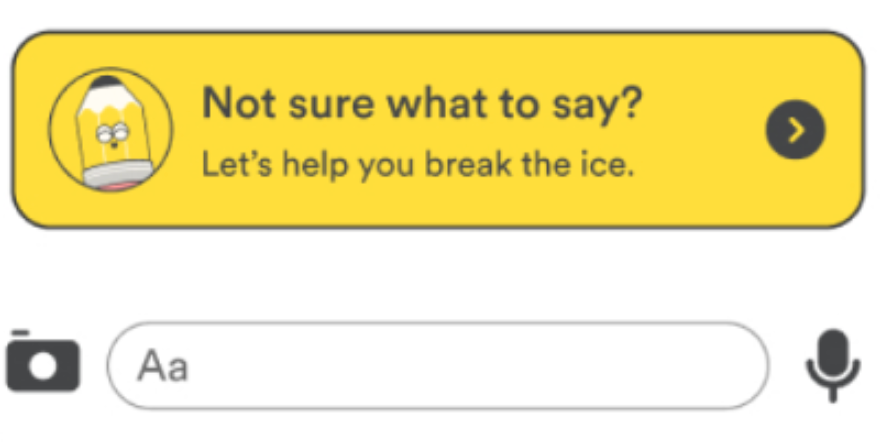
In all examples, the feature moves beyond basic content rewriting to increase the message’s effectiveness. Some do this by improving a user's quality of content based on best practices. Others do this by identifying unique characters that can be included in the message based on additional variables collected within the system. It even shows up as fewer words overall, increasing the message's efficiency.
Summarizing Content Overload
Summarization: While content rewriting remains a prevalent application of Generative AI, extracting actionable insights from voluminous content has rapidly gained traction as a vital use case. The most straightforward method is summarization, where significant amounts of data are processed to discern patterns and key points. LinkedIn’s article summarization feature exemplifies this, transforming lengthy posts and articles into digestible snippets, making complex information accessible at a glance.
Identifying Highlights: Similar to summarization but incorporating an element of judgment, features like Microsoft Team CoPilot’s call transcript summaries distill extensive discussions into essential bullet points, spotlighting pivotal moments or insights. Rippling AI and Greenhouse, both HR technologies, have released features that do the same with interviews. They can take the transcripts of interviews combined with the notes written by the interviewer to create summaries while highlighting the positives and negatives of each candidate. The same is being done in the B2B space with ServiceNow, which creates a collection of features to summarize and highlight patterns among support tickets. From there, they are expanding into two more emerging feature sets: report creation and advanced search.
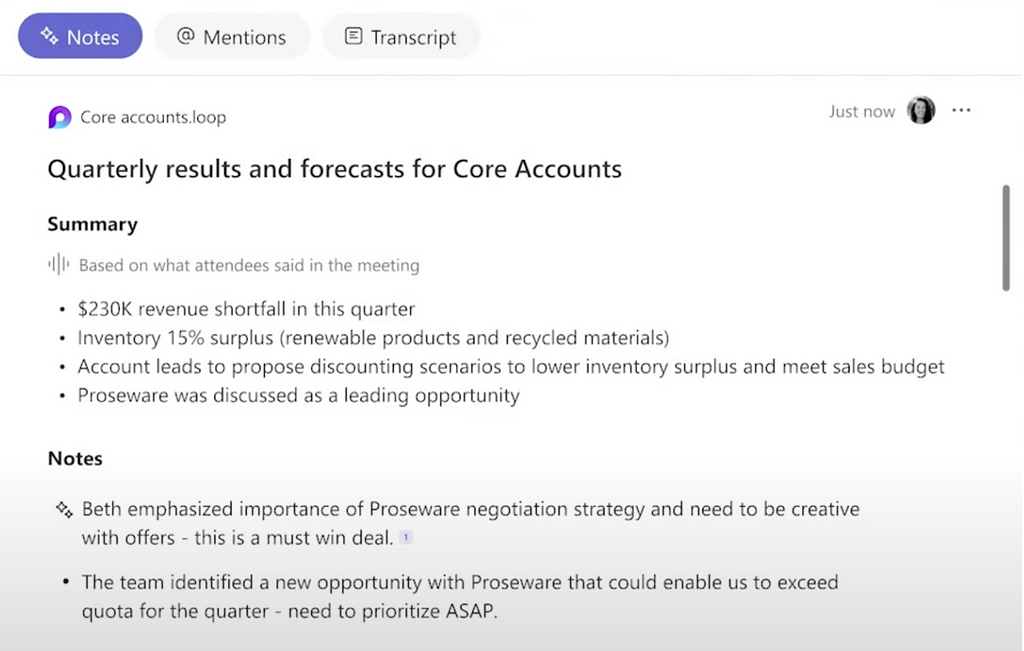
Report Creation: With summarized data, a natural next step, especially in SaaS products, is the creation of reports. By identifying the most important variables or common patterns, a product can auto-generate a deliverable for users to build upon. ServiceNow does this for customer service, just as Hubspot does for sales data. Tableau takes it further by automatically creating summaries based on trending data. Not only in B2B products, but Priceline has also recently launched a few GenerativeAI features, with the automatic creation of an itinerary being a leading example. This feature builds a plan for a user to adhere to during an upcoming trip. While still an early feature, there is much potential for further expansion and value-creation opportunities.
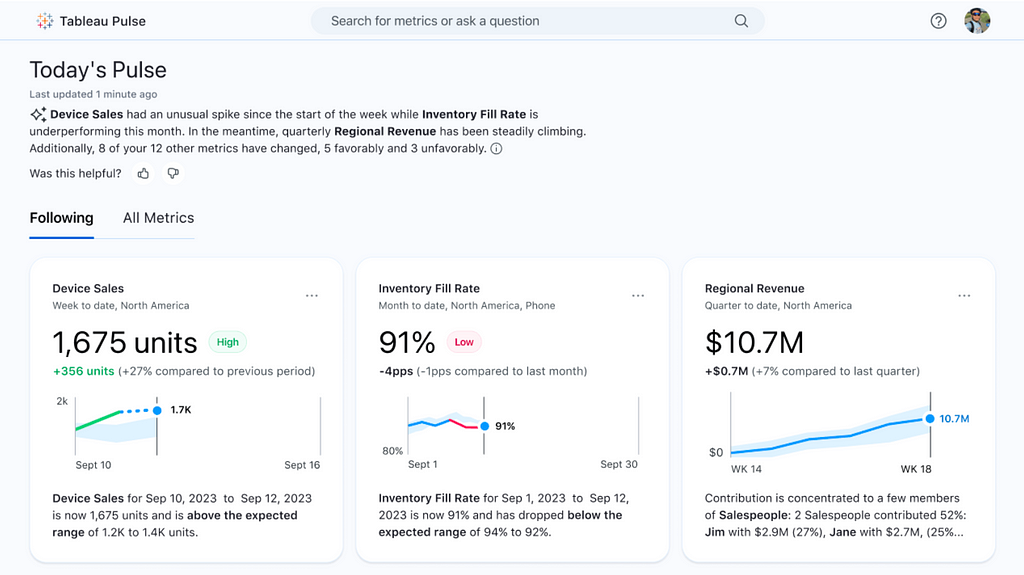
Advanced Search: The ability to ‘understand’ nuanced language through summarization extends naturally into advanced search functionalities. ServiceNow does this by enabling customer service agents to search tickets for recommended solutions and to dispel jargon used by different agents. ZenDesk does something similar by pulling up relevant information related to a service ticket. Concur takes the same format of pulling up relevant documents to an expense report, enabling employees to file their expense reports faster.
In a more traditional search fashion but with much more value, given the ability to rapidly summarize contents are the new features offered by Dropbox. Users can ask questions about the documents within their storage systems, quickly extracting insights without opening a variety of documents. Glean does the same by using an interactive chatbot so people can continue their line of questioning. The most innovative end of this spectrum allows for the inclusion of quantitative data, which Mixpanel is doing with its Spark AI. Users can ask questions about their data, searching for value among metrics.
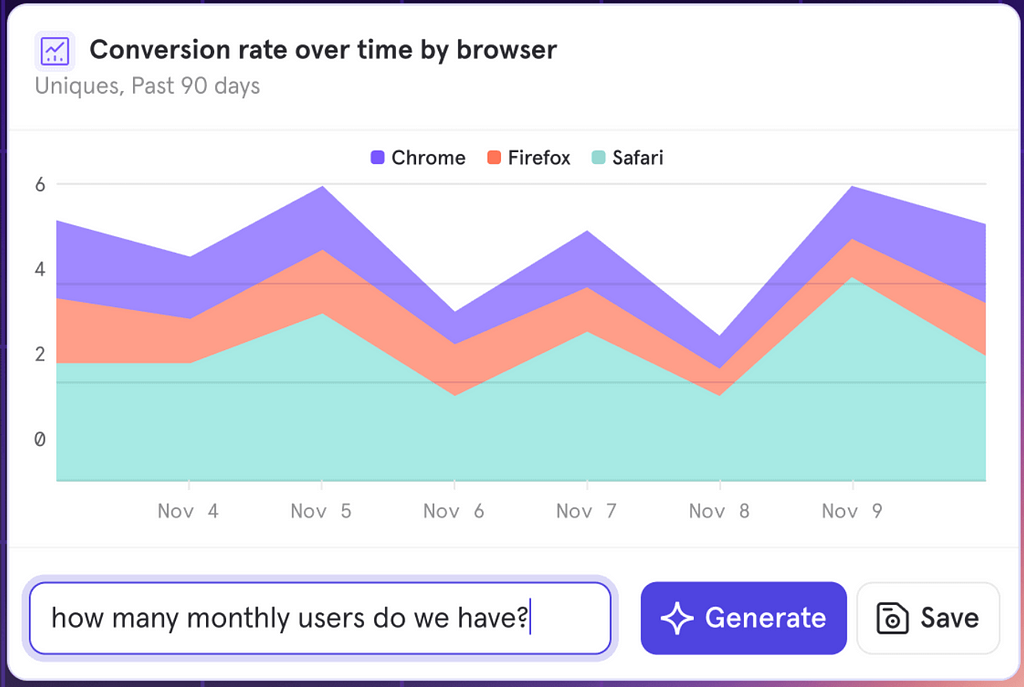
Insight Recognition: The final dimension of finding value in lots of emerging content is the identification of novel insights. Mixpanel and Tableau are already doing that along with a collection of AI-specific products. Gong, a sales enablement tool, has a new feature that identifies trends in what is working during sales calls. It uses GenAI to identify these patterns across similar but different words, triangulating around a central meaning that appears effective regardless of how it is exactly communicated to the potential customer. On the consumer side, Fitbit has begun to utilize users’ specific actions in combination with the corpus of users’ data to display personalized health insights. Combined with the tailoring of messaging, I imagine the natural next step will be to nudge the user to take personalized actions.
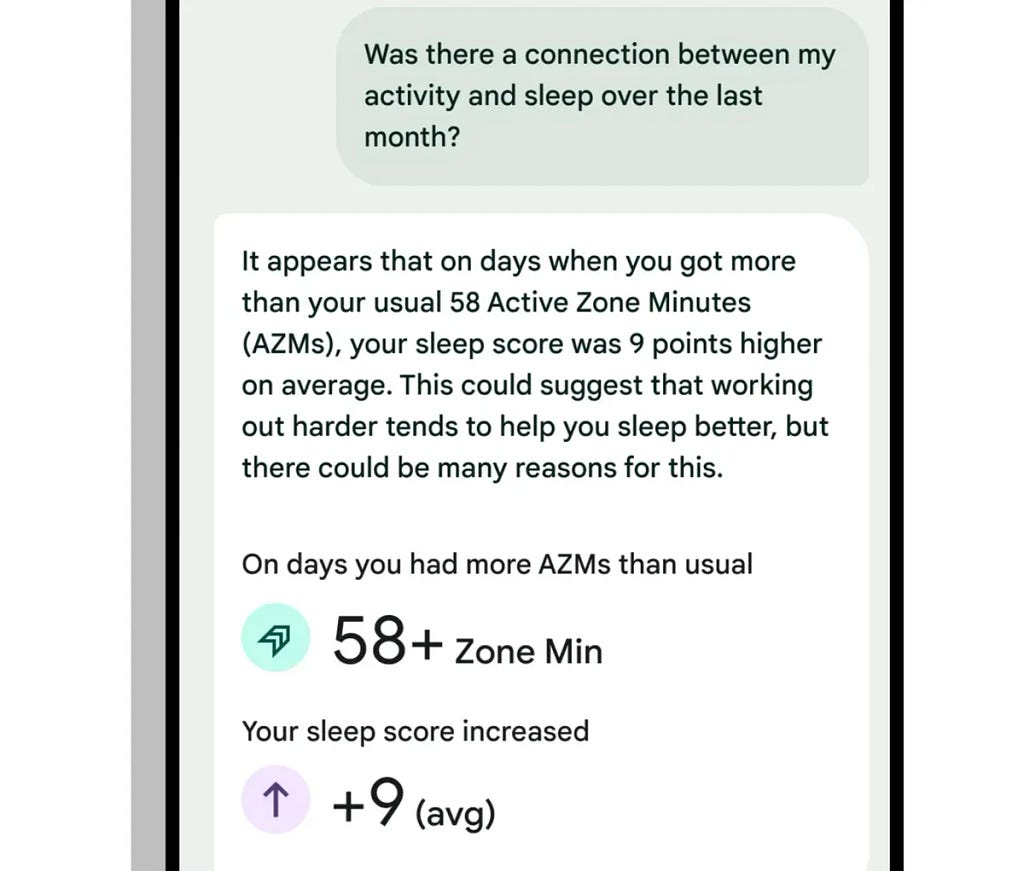
These design patterns demonstrate the multifaceted capabilities of summarization as a fundamental aspect of Generative AI. As experimentation continues, we can expect to see an expansion of these applications, with successful models being refined and replicated across industries. Just as with content rewriting, summarization and its many manifestations are but one of many burgeoning superpowers of Generative AI, paving the way for future innovations. Another superpowers that are just beginning to emerge is the ability to create evidence-backed predictions based on the collective knowledge that the foundational LLMs hold due to their training data.
Tapping into Collective Knowledge
Despite some controversies and unresolved issues, the undeniable reality is that large language models (LLMs) are trained on a vast corpus of human knowledge, covering a diverse range of data sources and content types. While these systems may not truly “understand” content in the human sense, they excel at recognizing patterns in human behavior. This capability underpins their effectiveness in role-playing specific personas, achieved by crafting prompts that guide the system to assume a desired stance.
Organizations are only beginning to explore this collective knowledge's potential in their product features. Rather than merely focusing on content creation or manipulation, emerging applications of these systems provide new perspectives and predict outcomes based on accumulated human experiences. The actual value of these applications lies not merely in enhancing efficiency but in augmenting effectiveness, enabling users to make more informed decisions. While still not widespread, I foresee this approach becoming a mainstay on company roadmaps, representing the next evolutionary step in AI application. Once an LLM generates a novel insight, the next logical question is, “What should I do with this information?” Using Generative AI to inform decisions based on the collective experiences and actions of others offers a powerful new tool for decision-making.
Scenario Planning: In professional settings and our personal lives, we frequently encounter situations that feel unique to us. However, others have likely navigated similar challenges. Generative AI can leverage this vast repository of experiences to offer guidance and predict potential outcomes. For instance, BetterUp’s Difficult Conversation Scenario Planner utilizes this technology to help users strategize for challenging interactions. By simulating different conversational paths, the tool reveals the potential effects of various approaches and allows users to anticipate aspects of the dialogue. In a B2B context, companies like AMEX are experimenting with Generative AI to forecast customer behaviors, aiming to refine financial planning processes.
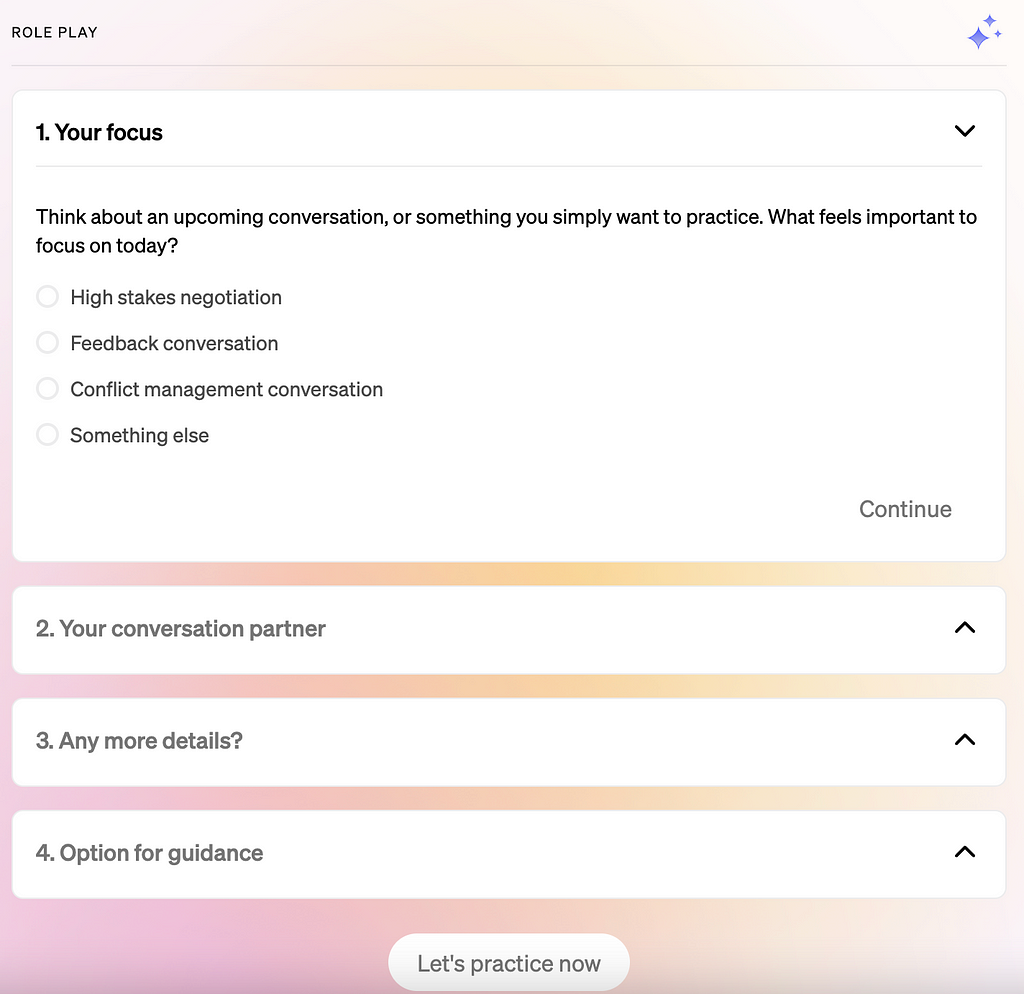
Empathy Building: Beyond scenario planning, these systems can deepen understanding between individuals. Our divisions often stem from a lack of understanding, and Generative AI, trained on a broad spectrum of perspectives, can serve as a bridge. For example, an experimental feature I observed allowed users to explore how they would react in a scenario and understand why others might choose differently. While full realization of this technology is on the horizon, LinkedIn’s current feature that suggests questions following posts hints at future possibilities. Users could query, “Why would someone take this perspective?” or “Why would this user feel comfortable sharing this?” Generative AI could then offer predictions that foster empathy among participants.

As we continue to unlock the capabilities of summarization and tapping into collective knowledge, these new generative AI applications not only promise to replicate knowledge but to contextualize and humanize it, potentially transforming how we interact with and understand each other.
The Evolution Continues
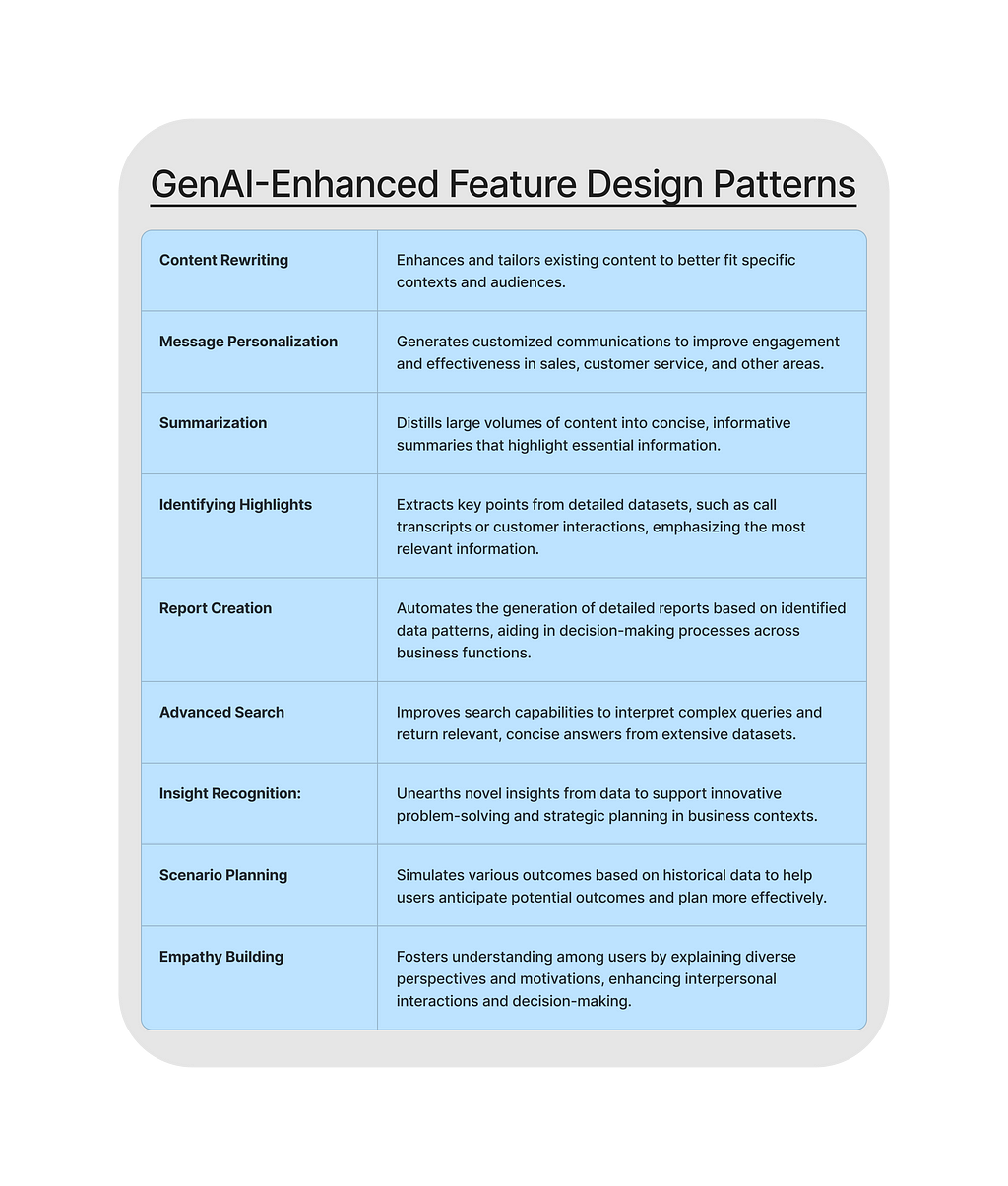
I have covered nine design patterns for AI-enhanced features emerging across the product landscape. All of this has occurred in less than a year! Looking forward, I am sure some of these will stick, and others will be overtaken by better applications. As entire AI-first products are built, it will be fascinating to see what angles are used and how many of these early features remain. What is sure is that the evolution will continue as teams learn how to build solutions that are fit for this novel technology.
In this exploration, I have highlighted nine distinct design patterns for AI-enhanced features that have proliferated across the product landscape — all within the span of less than a year. As we look to the future, some of these innovations will likely become staples while others may fade, surpassed by more sophisticated applications. The rise of AI-first products promises to introduce fresh perspectives and methodologies, potentially reshaping which of these early features remain relevant.
What remains certain is that the evolution of AI-enhanced features will persist as development teams continue to refine their approach to integrating this transformative technology. The key to future success lies in the ability to adapt and innovate, ensuring solutions are technologically advanced and deeply aligned with human needs and contexts. As we continue to navigate this exciting terrain, the principles of user-centered design will guide us toward creating functional tools and transformative experiences that redefine how we live and work. The next chapter of product design is being written today, and Generative AI is undeniably a leading character in this unfolding story.
The rise of Generative AI-driven design patterns was originally published in UX Collective on Medium, where people are continuing the conversation by highlighting and responding to this story.