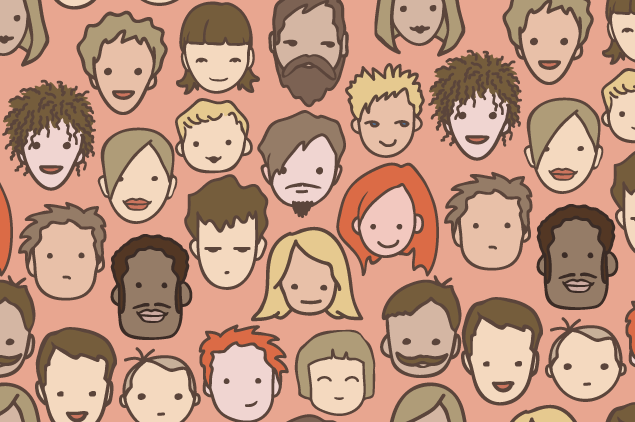
Much of our work as user experience designers is understanding the whys and why nots of our audiences’ decision making. In a previous post, I explored why people decide to switch or stay with products, focusing mainly on circumstances, the “where” and “when” of a job. The circumstances in which a job is done is an example of a “Job driver”. Job drivers are influences external to the job itself that cause people to take an action over another. These job drivers break down into three categories: the job actors' attitudes, background, and circumstances (Wunker, 2017).
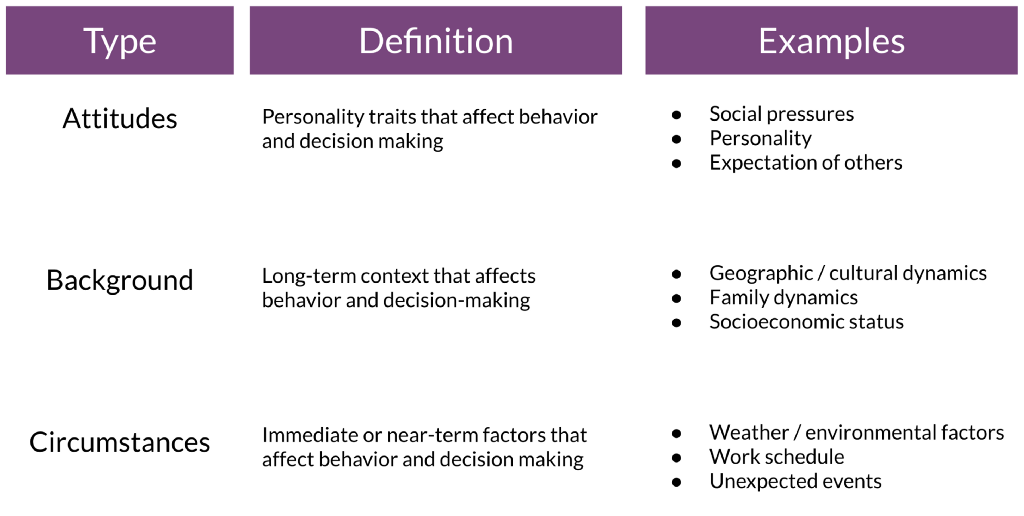
Attitude is a person’s positive or negative evaluation of place, person, situation, activity, etc (Eagly & Chaiken, 1993). For simplicity's sake, I will abstract these and call them “an object”. These attitudes split into three areas: affective (feelings), behavioral (the effect of the attitude on behavior), and cognitive (belief and knowledge) (Rosenberg & Hovland, 1960). I will be using this model to describe how understanding the interrelationships of these could start to provide both a human and outcome-driven mindset for our design teams and allow us to be much more deliberate with the types of features we pursue.
Our scenario
To frame this article I will be describing how these attributes might come together when designing for a complex behavior such as getting students (learners) to do additional work beyond what was assigned by the instructor. It is sometimes difficult to get them to slow down and put in the additional work needed to be successful in school. A large part of this is motivation. In this post, I will focus on attitudes and its impact on motivation. Gaining a deep understanding of this could help designers take a more strategic approach to design when attempting to nudge behavior patterns.
Affective (feeling, emotion)
Affect is the emotion and feeling that a student is experiencing at any given time. This could include negative emotions such as boredom, frustration, and anxiety, as well as positive emotions such as joy, interest, and passion.
Having positive affect has been shown to increase motivation, helping students to persevere when doing a task (Winne, 1998). Affect is also closely tied to personality traits, which can influence how individuals react in certain situations (Fetvadjiev, 2019).
In psychology, five broad dimensions (the ‘Big Five’) are commonly used in the research and study of personality. The nice thing about personality traits is that they tend to stay relatively stable throughout most of one’s lifetime (Chmielewski, 2013).
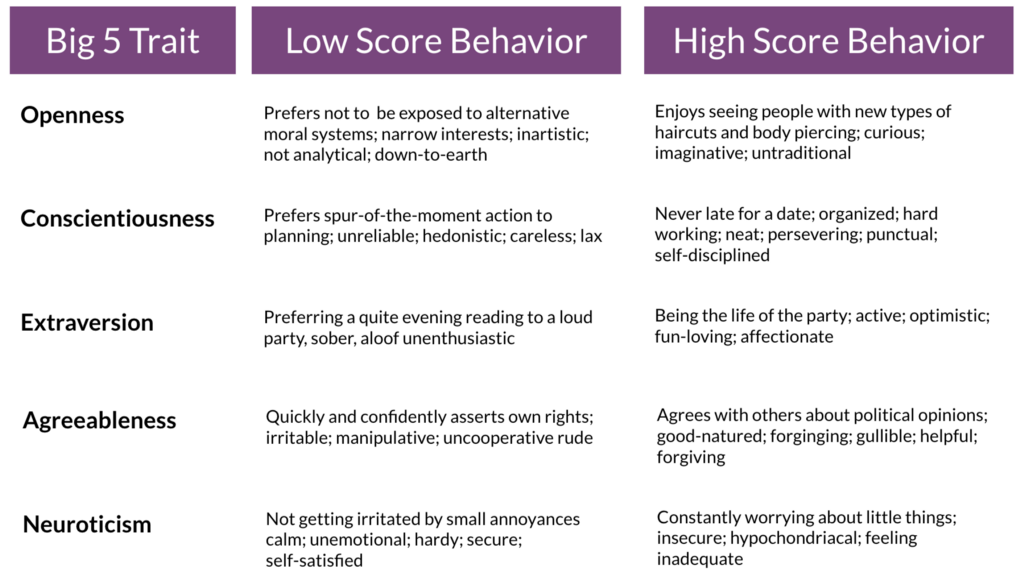
Using a similar thought process we could start narrowing down traits specific to learners. It is important to note, however, that learner traits may be influenced by cognitive and emotional aspects of attitude, meaning they could act one way in a composition class, and another in math class. Unlike personality traits that tend to be stable over long periods of time, some learner traits might be dependent on variable such as relevancy during their major. One example of a learner trait is how much of their motivation intrinsic versus extrinsic.
Intrinsic vs Extrinsic Motivation
There are several ways to describe motivation. One way is thinking about whether motivation arises from outside (extrinsic) or inside (intrinsic) an individual.(Tranquillo, 2016).
- Intrinsic: Students that are intrinsically motivated perform a behavior or engage in an activity because they find it rewarding such as fun, interesting, or they might be curious about the topic.
- Extrinsic: Students that are extrinsically motivated perform a behavior or engage in an activity because we want to earn a reward (such a grade) or avoid punishment (such as getting in trouble from their parents).
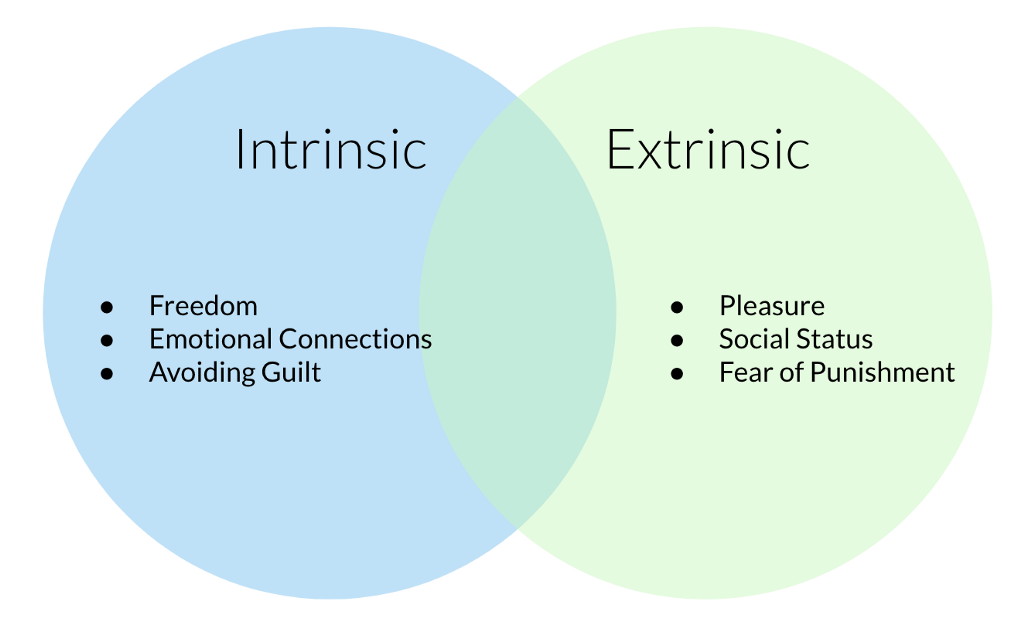
How might you get this information
- Listening Sessions (Indi Young)
- Field Observation
- Diary Studies
- Semi-structured qualitative interviewing
- Survey Diagnostic
Cognitive (knowledge, belief, performance, ability)
The cognitive aspects of attitudes involve a person’s belief/knowledge about an object, in this case, students’ personalized study plan. There are a couple ways cognitive factors might drive decision making in this example. Let’s start with Growth Mindset.
Growth vs Fixed Mindset (belief)
When learners have a growth mindset they believe that their talents can be developed through hard work, good strategies, and constructive feedback from others. In contrast, learners with fixed mindsets believe their talents are innate gifts. (Dweck, 2006)
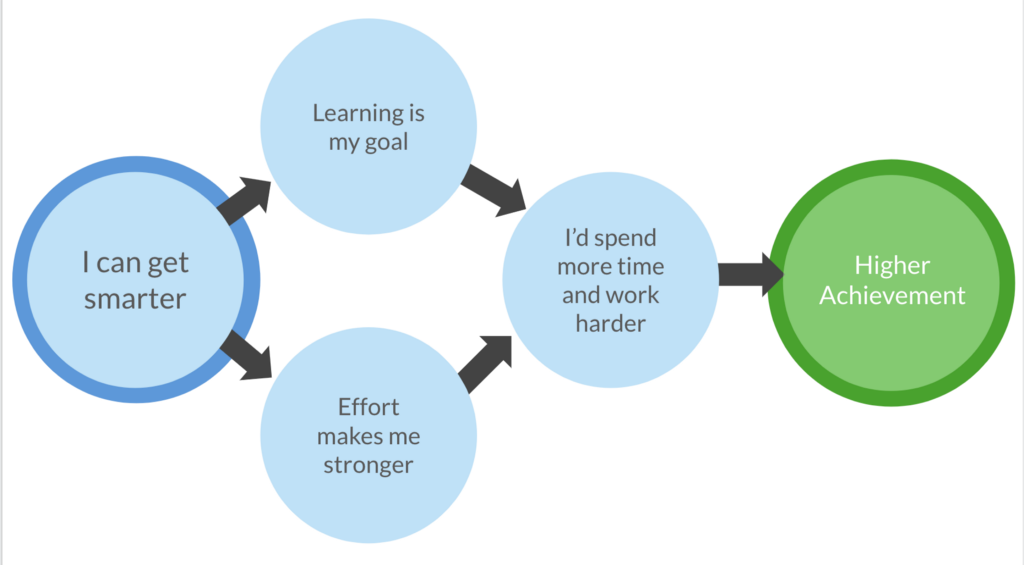
Here are a few other tensions that might influence a decision to use or not use optional study material.
Positive
- This targeted intervention is going to save me time by having me focus on the areas that matter.
- I watched a few of these and they were really helpful. It will save me time from hunting around in google.
- This report shows me where my weaknesses are. I could increase my grade by working on the weaknesses by using the study plan.
Negative
- I’ve already done a couple of the items in the plan and they are dull and boring. Because of that, I am pretty sure that the rest of these will be just as dull and boring. I will just use Google and find my own.
- I already know of another place that could help answer my question.
- I (think) that I already know this material all the material so doing additional work on something that I think I already know would be a waste of my time. Many students lack strong metacognitive skills, so helping to scaffold that can be helpful
Behavioral (actions, intentions)
Behavior is the way the attitude we have towards an object influences how we act or behave. For example, if we are designing personalized study material in an attempt to illicit self-regulated learning by students, it is fair to assume that unmotivated students will be less likely to engage with optional material that is not attached to their overall grade (extrinsic motivation) than highly motivated students.
As you can see in the example, there are possible correlations between both affective and cognitive attributes that mold a persons attitude which are externalized by their behavior towards an object or outcome. Assuming the desired outcome is “to better understand a topic”, we will need them to engage with our study material to get there. This will result a few actions. The optional material might be to ignore it completely, they might intend to work on it but never follow through, or they might complete the work.
Finding correlation of affect and cognition to behavior
In the case of our example, behaviors such as engagement with an activity could be quantified through field research and/or analytics. Let’s assume that we analyze our analytics on student behavior in our system and try to understand the frequency and duration at which a student opened and engaged with optional study plan material. You might decide to segment those students into categories such as:
- No Engagement: Never opened a resource, in last 7 days
- Low Intent: Opened resources, but never answered any questions, in last 7 days
- Low Engagement: Total questions answered< 5, in last 7 days
- Mid Engagement: Total questions answered < 10, in last 7 days
- High Engagement:Total questions answered > 10, in last 7 days
Once you know the types of behaviors you are expecting, you could start to understand what attitudes might be influencing certain students act differently than others by asking and answering a series of questions such as:
- Are students that perform better on their assignment more likely to engage with optional material? (Cognitive Driver)
- Are students that are grade-driven more likely to engage with optional material? (Affective Driver)
What do we do with all this data?
Once you have a dataset you could start clustering. Clustering is the task of grouping a set of objects in such a way that objects in the same group (called a cluster) are more similar (in some sense) to each other than to those in other groups (clusters). Creating these clusters with the information we’ve collected about our students above will allow us to start segmenting them into groups. By understanding our audience in this way we could start making decisions about our design.
Clustering activities come in many forms and fidelity. From affinity maps with sticky notes to machine learning algorithms. The techniques you use will depend on what you are trying to do.
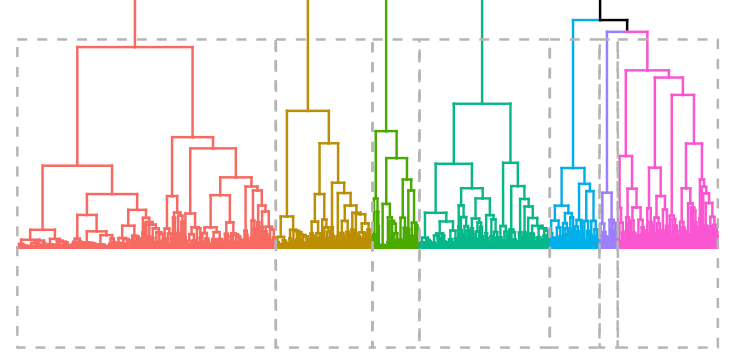
Describing the various clustering methods is outside of the scope of this article, but MesuringU has a great article that presents several methods.
How might we leverage this insight?
The question then becomes “how do we influence behavior in order to get students that are not motivated to engage with the material”. There are several behavior design frameworks, we will be looking at Fogg’s Behavioral Model (Fogg, 2020). This model gives a clue on how we might think about going about influencing behavior through the use of prompts.
Fogg argues that without giving a person an appropriate prompt (call-to-action) based on both their ability (do they believe that they could complete the task) and motivation (do they want to complete the task), you will have a difficult time eliciting the behavior you are trying to get your student to do. It is not enough to give any prompt, the prompt needs to be explicitly designed in a way where its goal is to either increase motivation or ability (Fogg, 2020). This means we need to know where on the Motivation and Ability spectrum our customers land. Let’s look at the 3 types of prompts and how they land on the chart:
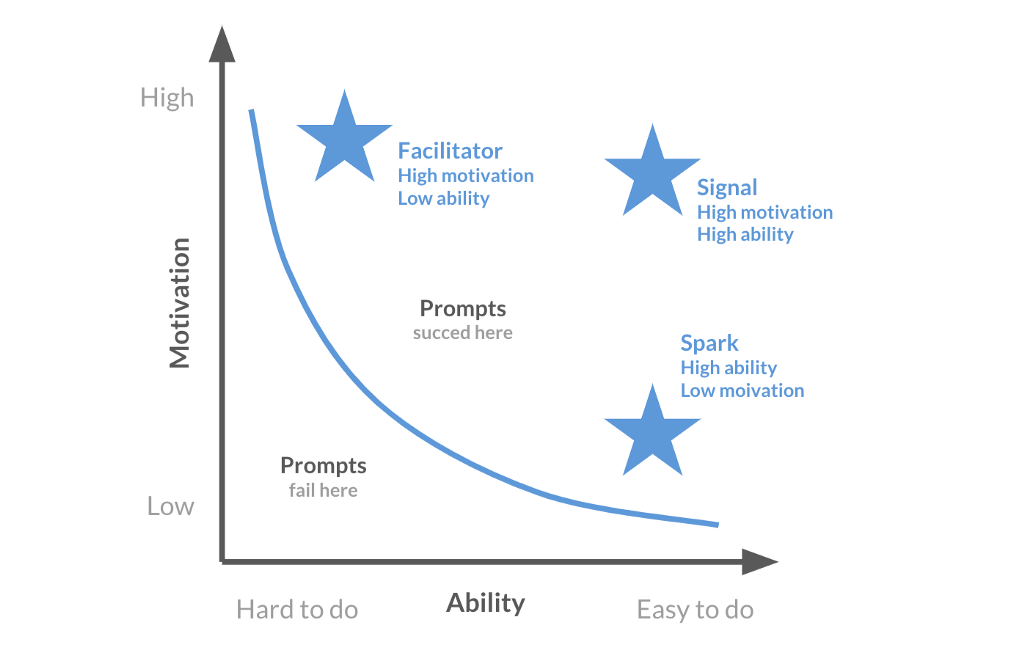
Spark
This user has high ability yet lacks motivation.
Self-determination theory states that having students set their own goals has been shown to help increase student motivation. In the example below the student is presented with their progress on the goal that they set for themselves and encouraging them to keep going.
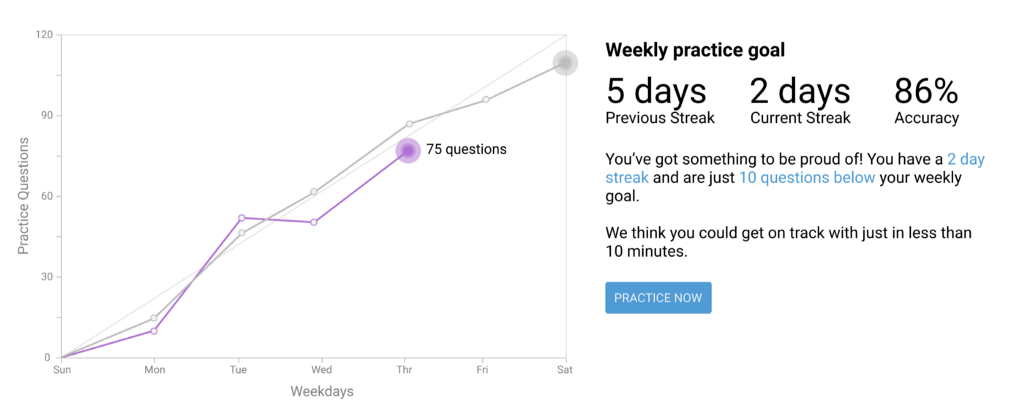
Facilitator
This user has low ability and high motivation.
The goal of designing for facilitators is to remove as much friction as you can to make the desired behavior easy to do. In the example below I have notified that student that there is a negative trend. Immediately to the right of it, there is a link to remediation. No
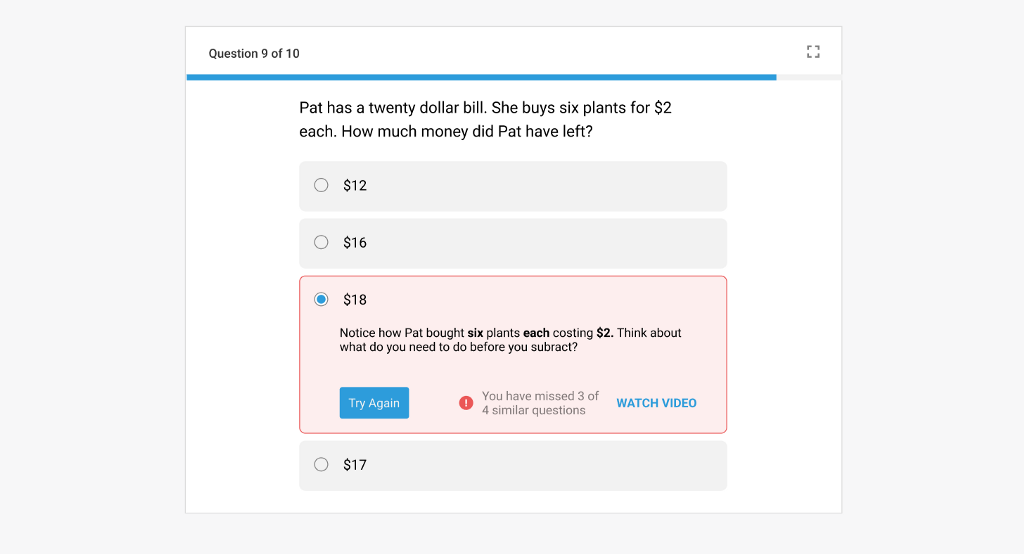
Signal
This user has both high ability and motivation.
There is not much needed for this user to do the task other than giving them a slight nudge. Be careful. not to jump solely to signal prompts These tend to be very light touch and are quite frankly easier to do. The reality however is that the probability that a large portion of your students are both motivated and have high ability is likely low.
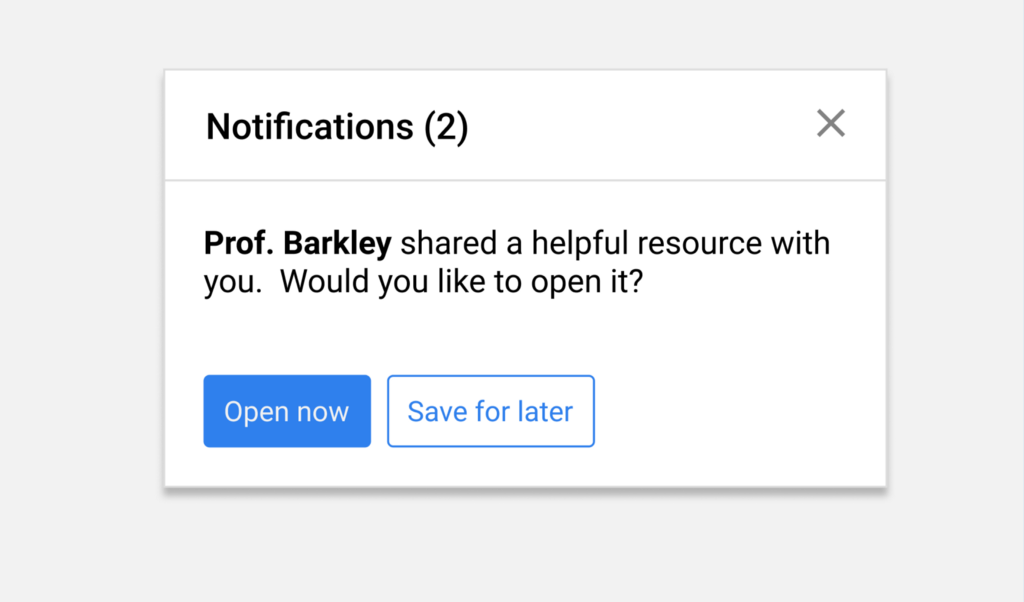
Timing is everything
It is not enough to design a good prompt. The prompt that you create needs to show up at the right time when it is most relevant. Communicate to users in situations that are the opportune moments for change.
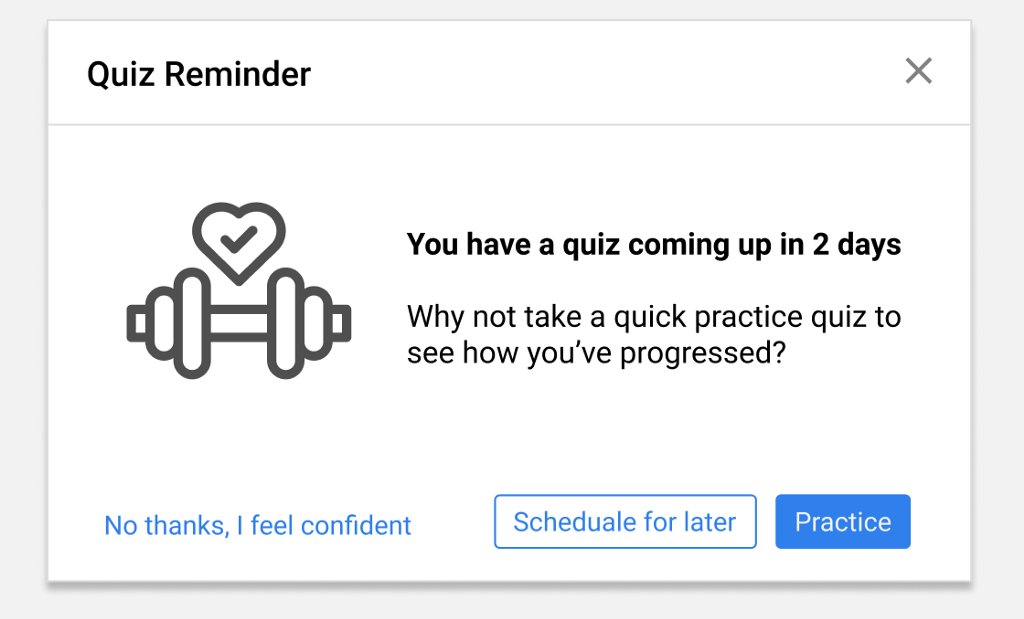
References
Chmielewski M.S., Morgan T.A. (2013) Five-Factor Model of Personality. In: Gellman M.D., Turner J.R. (eds) Encyclopedia of Behavioral Medicine. Springer, New York, NY. https://doi.org/10.1007/978-1-4419-1005-9_1226
Dweck, Carol. (2006). Mindset: The New Psychology of Success. Random House Publishing Group. Kindle Edition.
Eagly, A. H., & Chaiken, S. (1993). The psychology of attitudes. Harcourt Brace Jovanovich College Publishers.
Fetvadjiev, V.H. & He, J. (2019). The longitudinal links of personality traits, values, and well-being and self-esteem: A five-wave study of a nationally representative sample. Journal of Personality and Social Psychology, 117(2), 448–464.
Fogg, B. J.. 2020. Tiny Habits: The Small Changes That Change Everything. Boston: Houghton Mifflin Harcourt.
Rosenberg, M. J., Hovland, C. I., McGuire, W. J., Abelson, R. P., & Brehm, J. W. (1960). Attitude organization and change: An analysis of consistency among attitude components. (Yales studies in attitude and communication.). Yale Univer. Press.
Tranquillo J, Stecker M. Using intrinsic and extrinsic motivation in continuing professional education. Surg Neurol Int. 2016;7(Suppl 7):S197–9. doi:10.4103/2152–7806.179231
Winne, P. H., and Hadwin, A. F. (1998). “Studying as self-regulated learning,” in Metacognition in Educational Theory and Practice, eds D. J. Hacker, J. Dunlosky, and A. C. Graesser (Mahwah, NJ: Lawrence Erlbaum Associates Publishers), 27–30.
Wunker, Stephen. Jobs to Be Done: A Roadmap for Customer-Centered Innovation . AMACOM. Kindle Edition.
The ABCs of attitude-based archetypes in design was originally published in UX Collective on Medium, where people are continuing the conversation by highlighting and responding to this story.